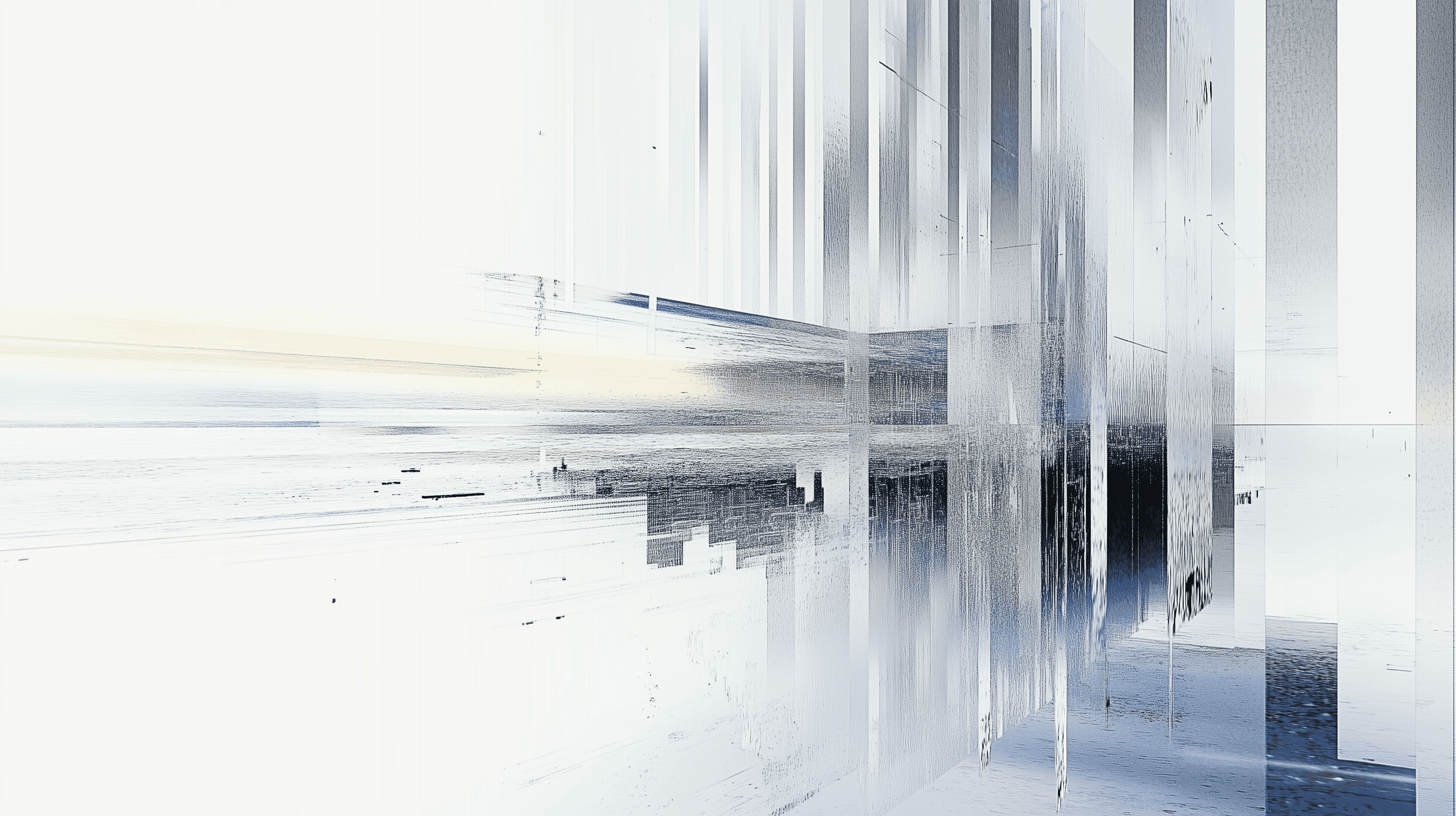
Evaluating language models has become increasingly complex as their capabilities rapidly evolve. Traditional benchmarks like GLUE and SuperGLUE quickly became obsolete as modern LLMs reached superhuman performance on basic language understanding tasks. This shifting landscape has created a critical challenge for teams building AI products: how do you meaningfully assess model performance across dimensions that actually matter for your applications?
This article maps the evolution from simple metrics to sophisticated evaluation frameworks like MMLU, HELM, and BIG-bench. We examine how these frameworks measure different capabilities—from factual knowledge and reasoning to conversation quality and instruction following—using both quantitative metrics and qualitative human judgment. The technical architecture behind each framework reveals important methodological differences that directly impact reported performance.
By understanding these evaluation methodologies, you'll be equipped to make more informed decisions about which models truly serve your product needs beyond headline benchmark scores. Move beyond leaderboard comparisons to develop evaluation strategies that align with real-world application requirements and business impact.
In this guide, we'll explore:
- 1The evolution and limitations of LLM evaluation approaches
- 2Technical architecture and implementation of leading benchmarks
- 3Mathematical foundations of assessment metrics
- 4Methods for evaluating reasoning, knowledge, and conversation skills
- 5Strategies for bridging benchmark performance to production results
Evolution of LLM evaluation frameworks
The landscape of language model evaluation has undergone significant transformation as LLMs have rapidly advanced in capabilities. Traditional benchmarks that once challenged models have quickly become outdated as models surpass human-level performance.
From simple metrics to multidimensional frameworks
Early evaluation frameworks like GLUE and SuperGLUE measured basic language understanding through tasks such as sentiment analysis and textual entailment. However, these benchmarks were soon outpaced. As one report notes, models "have outpaced the benchmarks to test for them," with recent models quickly reaching super-human performance on standard benchmarks.
This rapid saturation necessitated more comprehensive evaluation approaches. Simple metrics like accuracy or BLEU score proved insufficient for assessing the complex capabilities of modern LLMs. The evolution toward more sophisticated frameworks became essential as models continued to advance beyond the capabilities measured by traditional metrics.
Beyond traditional benchmarks
Modern evaluation frameworks now assess various dimensions simultaneously. These include:
- Knowledge tests: MMLU and TruthfulQA
- Reasoning frameworks: AI2 Reasoning Challenge (ARC) and LogiQA
- Technical evaluations: HumanEval for coding assessment
- Instruction following: MT-Bench
- Safety evaluations: HarmBench
Each framework addresses specific aspects of LLM performance that traditional benchmarks failed to capture. This diversification reflects the growing understanding that language models require multifaceted evaluation approaches to truly assess their capabilities.
Human evaluation complements automated metrics
Despite advancements in automated metrics, human evaluation remains crucial. Frameworks like Chatbot Arena provide subjective quality assessments that automated metrics cannot capture. Human evaluators assess nuances in:
- Coherence
- Relevance
- Fluency
This complementary approach provides a more holistic understanding of a model's capabilities and limitations. The balance between human judgment and computational metrics creates a more comprehensive evaluation landscape that better reflects real-world performance requirements.
Statistical significance in comparisons
Small differences in benchmark scores may not translate to meaningful real-world performance variations. Understanding statistical significance has become critical when comparing models.
Evaluating LLMs requires a balance of quantitative metrics and qualitative human judgment to truly understand their capabilities across different dimensions. This balanced approach helps organizations move beyond simplistic leaderboard comparisons to make informed decisions about which models best suit their specific needs.
Technical architecture of leading LLM benchmarks
Modern LLM evaluation frameworks employ sophisticated technical architectures to ensure consistent, reliable assessment of model capabilities. These benchmarks differ significantly in their methodological approaches, implementation details, and resource requirements. Understanding these technical foundations is essential for interpreting benchmark results meaningfully.
Methodological differences between MMLU, HELM, and BIG-bench
Each framework employs unique prompting strategies and evaluation methodologies to test different dimensions of language model capabilities. These methodological differences highlight the importance of understanding what each benchmark actually measures before drawing conclusions about model performance.
Benchmark framework implementations
The technical implementation of these frameworks varies significantly. EleutherAI Harness offers a unified, efficient architecture for benchmarking, enabling consistent evaluation across different models. This differs from the original implementations of benchmarks like BIG-bench, which often use bespoke evaluation scripts tailored to specific tasks.
These implementation differences can significantly impact reported scores. Minor variations in prompting, temperature settings, or sampling methods can lead to substantial performance variations, even when testing identical models on the same benchmark. This reality underscores the importance of standardized implementation practices when comparing benchmark results across different studies.
Computational requirements for comprehensive benchmarking
Comprehensive LLM benchmarking demands substantial computational resources:
- HELM's full evaluation suite: ~500 GPU hours per model
- BIG-bench (broader task range): Even more extensive resources
- Resource intensity varies based on model size
- Larger models demand exponentially more memory and computation time
The resource barrier creates challenges for smaller research teams and necessitates efficient evaluation frameworks to make benchmarking more accessible.
One small improvement has made benchmarking more inclusive: the adoption of tensor parallelism in evaluation frameworks, allowing distribution of computation across multiple devices. These advancements in resource optimization are crucial for democratizing access to comprehensive model evaluation.
Statistical significance calculation methods
Modern benchmarks implement sophisticated statistical methods to determine meaningful performance differences. HELM employs bootstrap resampling techniques to calculate confidence intervals, allowing for more reliable model comparisons.
Statistical significance in these frameworks often relies on paired tests across multiple task instances, rather than simple accuracy comparisons. This approach helps distinguish genuine performance improvements from random variation, particularly important when comparing models with similar capabilities. These methodologies provide a more rigorous foundation for model comparison than simple point estimates.
Anti-overfitting mechanisms
Benchmark architects have implemented several anti-overfitting mechanisms to prevent data contamination and ensure evaluations remain meaningful. These include:
- 1Dynamic dataset rotation in benchmarks like LiveBench, which refreshes evaluation data every six months
- 2Private evaluation sets with controlled access for critical assessments
- 3Adversarial filtering techniques that identify and remove examples potentially seen during training
- 4Automatic detection of memorization patterns versus genuine reasoning
These mechanisms are crucial as models grow larger and training datasets encompass more of the internet, making data contamination an increasingly significant challenge in accurate evaluation. The ongoing battle against benchmark contamination highlights the evolving nature of evaluation methodologies in response to ever-larger training datasets.
Metrics and mathematical foundations for model assessment
The mathematical frameworks underlying LLM evaluation provide the quantitative basis for comparing model performance. Understanding these foundations is essential for interpreting benchmark results and developing effective evaluation strategies.
Understanding evaluation metrics
Evaluation metrics form the mathematical backbone of assessing large language model (LLM) performance. Different metrics measure specific aspects of model outputs, with each capturing unique dimensions of quality.
Types of Metrics:
These metrics represent different mathematical approaches to the same challenge: quantifying the similarity between generated and reference text in a meaningful way. The diversity of metrics reflects the complexity of language evaluation, where no single mathematical approach can capture all aspects of quality.
Semantic similarity metrics
Traditional n-gram based metrics have significant limitations. They cannot capture linguistic nuances like paraphrasing, dependencies, or polysemy. More advanced semantic metrics address these gaps.
BERTScore leverages contextual embeddings to evaluate text by representing tokens in a high-dimensional vector space. Its mathematical foundation relies on cosine similarity between these vector representations:
- 1Each token is mapped to a contextual embedding vector
- 2Token-level matching uses cosine similarity to find optimal pairings
- 3Importance weighting applies inverse document frequency to prioritize informative tokens
Correlation with Human Judgment:
This superior alignment with human evaluation illustrates how advanced mathematical approaches can provide more meaningful assessment of language quality.
Statistical validation methods
Reliable evaluation requires statistical validation beyond simple scoring. Confidence intervals provide a range where the true value likely falls, helping distinguish meaningful performance differences from random variation.
When comparing models, statistical significance testing determines whether observed differences represent genuine performance gaps. Common approaches include:
- Bootstrapping: Resampling techniques that generate distributions of metric scores
- Non-parametric tests: Wilcoxon signed-rank tests for comparing paired observations without assuming normal distribution
- Correlation analysis: Spearman's ρ, Pearson's r, and Kendall's τ measure alignment between automated metrics and human evaluations
The strength of these correlations varies greatly by task and metric. For instance, SapBERT Score shows a Spearman correlation of 0.185 with human evaluations, outperforming ROUGE-L (0.113) in clinical text evaluation. These statistical validation techniques provide essential context for interpreting benchmark scores and understanding their reliability.
Log probability for hallucination detection
Detecting hallucinations requires specialized mathematical approaches. Log probability calculation leverages a model's confidence in its predictions.
The perplexity metric, defined as the exponentiated average negative log-likelihood, measures how "surprised" a model is by text. Lower perplexity suggests higher confidence. Mathematically:
Where N is the sequence length and P(xi|x<i) is the probability of token xi given preceding tokens.
By analyzing token-level probabilities, we can identify potential hallucinations when models generate high-confidence outputs not supported by source texts. This mathematical approach enables quantitative measurement of a previously subjective concept. The application of probabilistic methods to hallucination detection represents an important advancement in evaluation methodology.
Cost-benefit analysis of evaluation approaches
Different evaluation approaches present varying trade-offs:
The optimal evaluation strategy combines multiple metrics to provide a comprehensive assessment across different dimensions of model performance. This mathematically informed, multi-faceted approach enables more reliable and nuanced evaluation than any single metric alone. Understanding these trade-offs helps organizations design evaluation frameworks that balance resource constraints with assessment quality.
Reasoning and knowledge evaluation methods
Assessing how language models reason and apply knowledge presents unique challenges that require specialized evaluation approaches. These frameworks focus on measuring logical abilities, distinguishing reasoning from memorization, and evaluating factual consistency.
AI2 Reasoning Challenge for multi-step reasoning
AI2 Reasoning Challenge (ARC) provides a robust framework for measuring an LLM's ability to perform multi-step reasoning tasks. This benchmark consists of grade-school science questions requiring logical inference beyond simple pattern matching. Models must demonstrate step-by-step problem-solving capabilities rather than relying on pure memorization or pattern recognition.
Complementary Frameworks:
- HumanEval: Focuses on code generation
- Evaluates: Translation of reasoning into functional code
- Measures: Capability to implement logical solutions to programming problems
These frameworks test different dimensions of reasoning ability, providing a more comprehensive assessment of an LLM's logical capabilities.
Distinguishing knowledge recall from reasoning
A critical challenge in LLM evaluation is determining whether models are genuinely reasoning or simply recalling memorized information.
Methodological approaches to this distinction include:
- 1Controlled testing with novel problems that couldn't have appeared in training data
- 2Presenting questions that require applying known concepts to unseen scenarios
- 3Using benchmarks like LogiQA that target this distinction specifically
These specialized frameworks help reveal whether models are truly reasoning through problems or merely regurgitating training data patterns.
Frameworks for factual consistency evaluation
TruthfulQA stands as a primary framework for evaluating factual consistency in LLMs:
- Consists of 817 questions across 38 categories
- Designed to assess whether models generate truthful answers rather than plausible-sounding falsehoods
- Specifically targets "imitative falsehoods" – incorrect answers that mimic common misconceptions found in training data
TruthfulQA helps identify how likely models are to produce hallucinations when responding to questions. By focusing on common misconceptions, this framework reveals how effectively models distinguish factual information from popular but incorrect beliefs.
Parametric vs. retrieval-augmented evaluation
Evaluation methodologies typically follow two distinct approaches:
Understanding the differences between these approaches helps contextualize benchmark results within practical application scenarios.
Statistical techniques for hallucination detection
Detecting hallucinations – fabricated information presented as factual – requires sophisticated statistical techniques. Common approaches include:
- Comparing model outputs against verified knowledge bases
- Measuring consistency across multiple generations of the same response
- Reference-free methods analyzing linguistic patterns associated with hallucinations:
• Unusually high confidence in speculative statements
• Semantic inconsistencies within generated text
These statistical approaches provide quantitative frameworks for measuring a phenomenon that otherwise depends on subjective assessment.
Benchmark design for isolating reasoning abilities
Effective benchmark design is crucial for accurately measuring reasoning capabilities. The most reliable benchmarks incorporate several key principles:
- 1Solution path annotation using techniques like depth-first search decision trees
- 2Generalization tests assessing whether models can apply reasoning skills to novel domains
- 3Human verification of reasoning steps to validate benchmark effectiveness
Tools like ToolLLaMA demonstrate that well-designed benchmarks can evaluate out-of-distribution performance, revealing how models handle unfamiliar contexts that require similar reasoning patterns. The combination of automated assessment and human verification creates a more comprehensive picture of reasoning capabilities.
Conversational and instruction-following assessment frameworks
As LLMs increasingly power interactive applications, evaluating their conversational abilities and instruction-following capabilities has become essential. Specialized frameworks address these interactive dimensions through both automated and human-centered approaches.
MT-Bench: evaluating multi-turn capabilities
MT-Bench is designed to test LLMs' ability to sustain multi-turn conversations:
- 80 multi-turn questions across 8 categories:
• Writing
• Roleplay
• Extraction
• Reasoning
• Math
• Coding
• STEM
• Social science - Two-turn structure: open-ended question followed by related follow-up
- Uses LLM-as-a-judge methodology (GPT-4 scores responses on 1-10 scale)
By focusing on multi-turn interactions, MT-Bench addresses a critical dimension of real-world conversational applications that single-turn evaluations miss.
Chatbot Arena: A competitive evaluation environment
Chatbot Arena offers a crowd-sourced evaluation platform where:
- 1Users interact with two anonymized LLM-powered chatbots simultaneously
- 2After submitting a prompt, users receive responses from both models
- 3Users vote for the better response
- 4Models' identities remain hidden until after voting
- 5Position bias is reduced through randomized response ordering
This platform generates win rates and battle counts between models, creating a competitive leaderboard that reflects real-world user preferences. The methodology effectively captures qualitative aspects of model performance that automated metrics might miss. This human-centered approach provides valuable insights into subjective qualities like helpfulness, naturalness, and overall user satisfaction.
Instruction-following metrics
The Decomposed Requirements Following Ratio (DRFR) provides a structured approach to measuring how well LLMs adhere to specific instructions. This metric breaks down complex requests into individual requirements and evaluates compliance with each component.
These frameworks address key evaluation challenges through:
- 1Statistical mitigation of position bias using randomized presentation
- 2Context retention assessment in multi-turn conversations
- 3Quantitative analysis of instruction adherence
By providing structured metrics for instruction following, these approaches enable more precise measurement of how well models understand and execute user requests - a critical capability for practical applications.
Balancing human and automated evaluation
Comparison of Evaluation Approaches:
Human evaluators excel at identifying nuanced issues with cultural context and practical usefulness that automated systems often miss. This hybrid approach provides the most reliable assessment of conversational and instruction-following capabilities. Finding the right balance between scalable automated evaluation and insightful human judgment remains a key challenge in conversational AI assessment.
Human Assessment Frameworks like Chatbot Arena complement automated benchmarks by capturing subjective quality judgments that are difficult to quantify but essential for real-world applications. This complementary relationship between different evaluation methodologies creates a more comprehensive understanding of model performance.
Connecting benchmark and production performance
Evaluating LLMs on benchmarks alone often fails to predict real-world utility. Research shows strong correlations exist between certain benchmarks and human evaluations, yet this relationship varies significantly across tasks and domains.
Organizations should design evaluation frameworks that reflect actual use cases rather than relying solely on leaderboard scores. As one researcher notes, "Benchmarks shape a field, for better or worse. Good benchmarks align with real applications, but bad benchmarks do not." This perspective highlights the importance of connecting abstract metrics to concrete business and user outcomes.
Creating representative evaluations
Continuous evaluation programs provide more meaningful insights than one-time assessments. Effective approaches include:
Testing Dimensions:
- In-domain capabilities
- Out-of-domain generalization
- Performance across multiple languages
- Environmental variable control (hardware differences)
Single metrics rarely tell the complete story. Instead, develop fine-grained evaluations that examine performance across different dimensions. This multifaceted approach provides a more comprehensive understanding of how models will perform in production environments with diverse user needs.
Connecting metrics to business impact
Benchmark results must translate into product impact through frameworks that link technical metrics to:
- 1User experience indicators
- 2Operational costs
- 3Real-world capabilities
For production deployments, measure performance under sustained load conditions that replicate actual usage patterns. This connection between technical performance and business outcomes ensures that evaluation efforts directly inform product decisions and development priorities.
Focus on the long tail
Rather than optimizing for average performance, organizations should care more about the worst-case scenarios. The true test of an LLM occurs at the edges of the distribution where improvements matter most for specialized applications.
Key Considerations:
- Statistical significance testing becomes increasingly important as the gap between models narrows
- Small benchmark differences may not translate to meaningful real-world performance variations
- Focus on challenging edge cases and reliability under varied conditions
- Develop robust applications that deliver consistent value to users
Human evaluations remain essential to complement automated benchmarks, especially for capturing subjective quality assessments that technical metrics might miss. This balanced approach to evaluation creates a more comprehensive understanding of model capabilities and limitations in real-world scenarios.
Conclusion
Effective LLM evaluation requires looking beyond headline benchmark scores to understand what specific capabilities matter for your product. As we've seen, the evaluation landscape has evolved from simple metrics to multidimensional frameworks that assess knowledge, reasoning, conversation skills, and instruction following—each requiring different methodological approaches.
Key takeaways for implementation:
- Adopt a hybrid evaluation strategy combining automated metrics with human assessment
- Use statistical validation methods (confidence intervals, significance testing) when comparing similar models
- Design evaluation frameworks that directly reflect your use cases
- Focus on the long tail of edge cases that impact user experience
For product teams, this means designing evaluation frameworks that directly reflect your use cases rather than optimizing for generic leaderboard performance. Focus on the long tail of edge cases where model behavior significantly impacts user experience. For engineers, understanding the mathematical foundations of metrics like perplexity and BERTScore enables more precise measurement of model capabilities and limitations.
Ultimately, the greatest business value comes from establishing continuous evaluation programs that connect technical performance to actual user outcomes. By bridging benchmark and production performance, you can make more informed decisions about model selection, fine-tuning strategies, and development priorities that deliver genuine product impact.