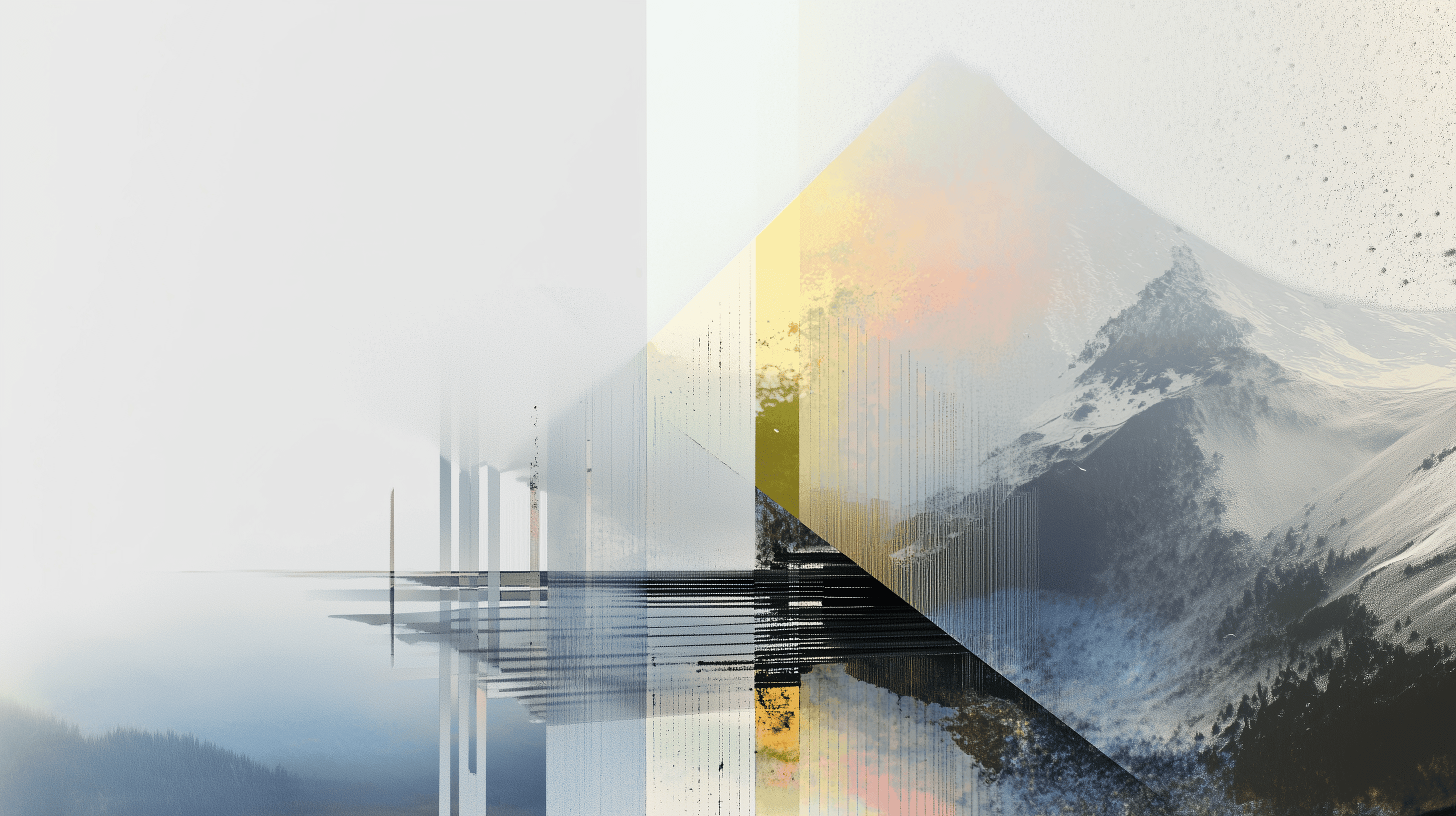
Batch inference represents a critical optimization technique for LLM-powered products, processing multiple requests simultaneously rather than sequentially. This approach can slash costs by up to 80% while significantly boosting throughput - a game-changer for teams managing production AI systems. The difference between efficient and inefficient batch processing often determines whether your LLM application remains financially viable at scale.
This guide explores both the theoretical foundations and practical implementation details of batch inference. We'll examine the memory-bound nature of LLM operations, dynamic batching architectures, and specific techniques like PagedAttention that dramatically improve resource utilization. These approaches address the fundamental challenge in LLM operations: memory bandwidth limitations rather than computational power.
By implementing the strategies outlined here, you'll achieve substantially higher throughput on existing hardware, reduce inference costs, and maintain better performance under varying workloads. These optimizations directly translate to improved economics for AI-powered features in your product.
Key Topics:
- 1
Fundamentals of LLM batch inference
Understanding prefill vs. decode phases - 2
Dynamic batching strategies
Moving beyond static approaches to continuous optimization - 3
Determining optimal batch size
Balancing throughput, latency, and hardware constraints - 4
Memory management techniques
KV cache optimization, quantization, and FlashAttention - 5
Variable-length input processing
Handling diverse content efficiently
TL;DR: Batch inference processes multiple LLM requests simultaneously instead of sequentially, reducing costs by up to 80% while boosting throughput. The key techniques include continuous batching, PagedAttention for memory optimization, and dynamic batch sizing (32-64 is optimal for most systems). These optimizations directly impact which AI features are economically viable at scale.
Fundamentals of LLM batch inference
Let's begin by exploring the core principles that make batch inference so powerful for LLM applications and the key components that drive its efficiency.
Batch inference in Large Language Models (LLMs) is a processing approach that groups multiple requests together for simultaneous execution. This method significantly improves computational efficiency and resource utilization compared to processing individual requests sequentially.
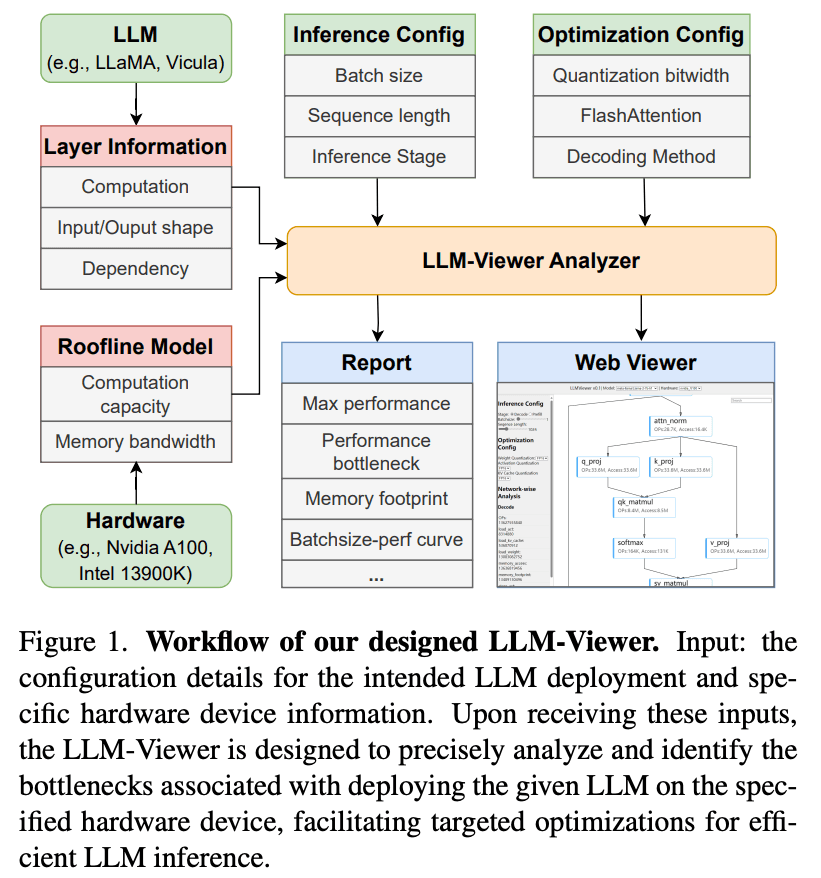
LLM-Viewer Analysis Framework. A diagnostic tool that analyzes model parameters, hardware specifications, and configuration settings to identify performance bottlenecks and optimal batch sizes for efficient LLM inference.
Source: LLM Inference Unveiled: Survey and Roofline Model Insights
Understanding the LLM inference process
LLM inference involves two distinct phases with different computational characteristics:
Prefill phase
During the prefill phase, the model processes an entire prompt at once to generate the first token. This phase is compute-bound, efficiently utilizing GPU resources through parallel token processing.
Decode phase
The decode phase generates subsequent tokens one at a time in an autoregressive manner. This phase is memory-bound rather than compute-bound. Loading model parameters from GPU memory consumes more time than the actual computation tasks performed by GPU cores.
This memory-bound nature makes batch processing particularly effective for improving overall throughput.
Key benefits of batch processing
Batch inference offers substantial advantages for non-real-time applications:
- 1Improved GPU utilization by processing multiple requests simultaneously
- 2Higher throughput with the same hardware resources
- 3Greater cost-efficiency compared to online inference (up to 70-80% cost reduction)
- 4Optimal resource allocation for background processing tasks
Batch size optimization
The optimal batch size represents a critical optimization parameter that balances several factors:
Studies show that increasing batch size from 1 to 64 can dramatically boost tokens processed per second. However, exceeding this threshold may risk system overload and diminishing returns.
KV caching and memory management
Key-Value (KV) caching is essential for memory optimization during batch inference. This technique stores intermediate computation results to avoid redundant processing while generating tokens.
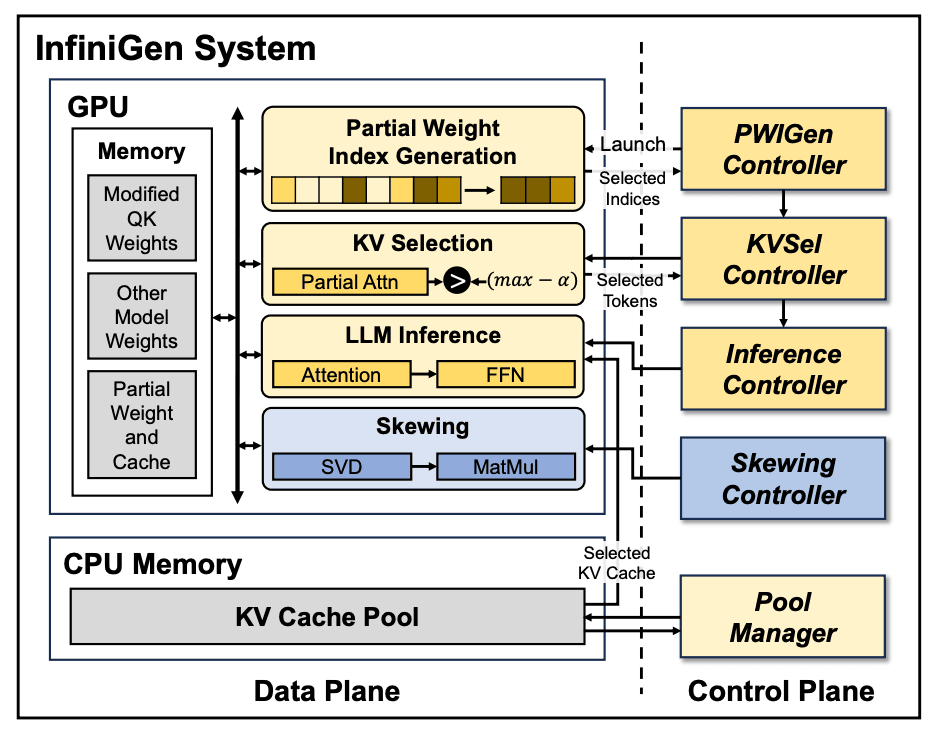
InfiniGen System Architecture. A memory-optimized inference system with dedicated KV cache management components that separate data and control planes for efficient token generation during batch inference.
Source: InfiniGen | Efficient Generative Inference of Large Language Models with Dynamic KV Cache Management
Effective KV cache management strategies include:
- 1
Pruning
Removing outdated or unnecessary cache entries - 2
Compression
Storing cached values in lower precision formats - 3
Sharing
Allowing multiple requests to use the same cached values when applicable
Memory bandwidth, rather than computational power, is typically the primary bottleneck in LLM inference.
Implementing efficient batch inference requires careful workload analysis, strategic scheduling, and continuous monitoring of batch efficiency metrics to maintain optimal performance. These fundamentals provide the foundation for more advanced batching strategies we'll explore in the following sections.
Dynamic batching strategies and implementation architecture
Building on our understanding of LLM inference fundamentals, we now turn to more sophisticated batching approaches that maximize resource utilization and significantly boost performance.
Understanding batching in LLM inference
Large language models (LLMs) are often memory-bound rather than compute-bound during inference. The time spent loading model parameters into GPU memory significantly exceeds the actual inference time. This makes batching—processing multiple requests simultaneously—critical for maximizing computational efficiency and throughput.
LLM inference consists of two key phases: the prefill phase (processing the input prompt) and the decode phase (generating new tokens). While the prefill phase is compute-bound and processes all input tokens in parallel, the decode phase is memory-bound, processing just one token at a time. This creates different optimization opportunities depending on the phase.
Static vs continuous batching
Static batching limitations
Traditional static batching maintains a fixed batch size throughout the entire inference process. When using static batching, sequences that finish early must wait for the longest sequence in the batch to complete before the GPU resources can be reallocated. This results in significant GPU underutilization, especially when generation lengths vary widely.
As sequence length variance increases, static batching performance drops dramatically—in some benchmarks, throughput plummets to as low as 81 tokens/second with high variance workloads.
Continuous batching advantages
Continuous batching (also known as dynamic batching or iteration-level scheduling) addresses these inefficiencies by:
- 1Replacing completed sequences with new ones at each iteration
- 2Dynamically adjusting batch composition during processing
- 3Allowing immediate injection of new requests when space becomes available
A key innovation in continuous batching systems is that they make scheduling decisions at the token level rather than the request level. When a sequence emits an end-of-sequence token, a new sequence immediately takes its place, significantly improving GPU utilization.
PagedAttention: Optimizing memory management
PagedAttention, implemented in systems like vLLM, introduces OS-inspired memory management techniques to further improve continuous batching:
- 1Dynamic memory allocation: Instead of pre-allocating fixed memory chunks, PagedAttention allocates memory in non-contiguous blocks as needed
- 2Logical/physical separation: Creates a mapping between the virtual view of the KV cache and physical memory blocks
- 3Reduced fragmentation: Eliminates up to 23x memory wastage compared to ahead-of-time allocation schemes
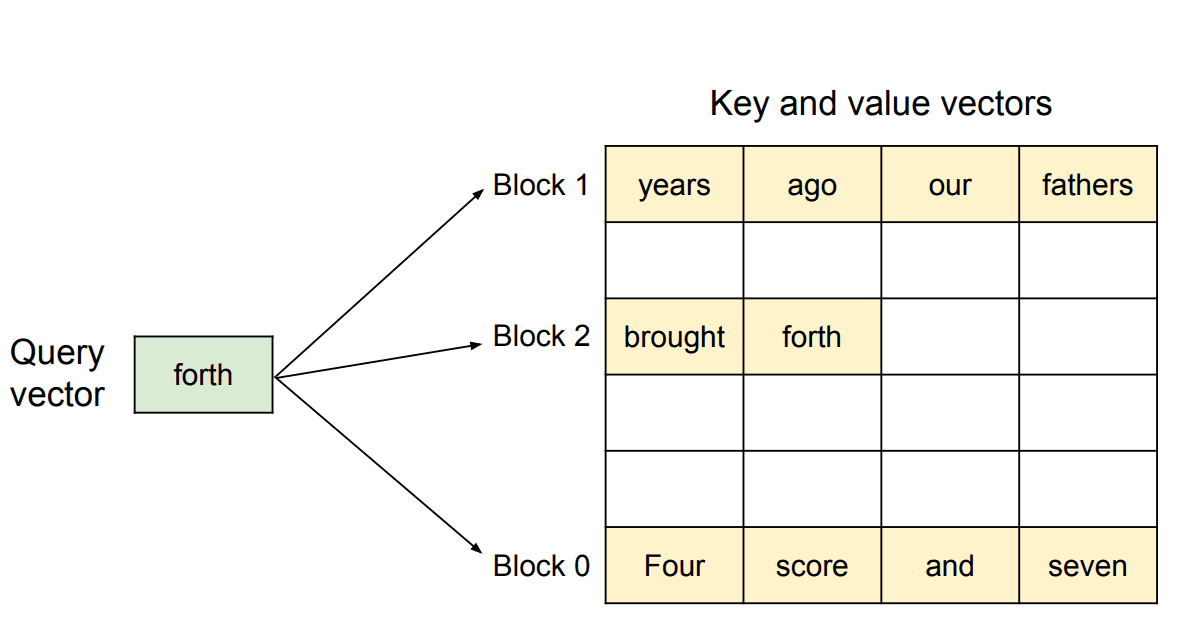
PagedAttention Block Allocation. Illustration of PagedAttention's non-contiguous memory management, showing how a query vector accesses relevant key-value tokens across separate memory blocks rather than requiring contiguous allocation.
Source: Efficient Memory Management for Large Language Model Serving with PagedAttention
This approach enables massive memory savings as most sequences won't consume their entire context window, translating directly to higher batch sizes and improved throughput.
Implementation architecture
Modern continuous batching systems are typically structured with:
The architecture must balance two competing priorities:
- Prefill-prioritizing scheduling: Eagerly adds new requests to maximize throughput but can introduce latency spikes
- Decode-prioritizing scheduling: Prioritizes ongoing token generation for smoother performance but potentially lower throughput
Performance benchmarks
Benchmarks show continuous batching dramatically outperforms static batching:
- Up to 23x throughput improvement using continuous batching with PagedAttention (vLLM)
- 8x improvement over naive batching when using continuous batching with Ray Serve or Hugging Face's text-generation-inference
- 4x improvement with optimized model implementations like NVIDIA's FasterTransformer
As workloads become more saturated (higher QPS), the performance gap between different batching strategies narrows, but continuous batching maintains its advantage, especially when enhanced with memory optimizations.
Implementation considerations
When implementing continuous batching, several parameters require tuning:
- 1
Maximum batch size
How many requests the model can process simultaneously - 2
Waiting-served ratio
The ratio of requests waiting for prefill to those waiting for end-of-sequence tokens - 3
KV cache management strategy
How to handle memory for the growing key-value cache during generation
Most production systems benefit from continuous batching, but there are scenarios where alternatives might be preferred. For low-QPS environments, dynamic batching can sometimes outperform continuous batching, and offline batch inference workloads might achieve better throughput with static batching by avoiding scheduling overhead. With these strategies in mind, determining the right batch size becomes the next critical consideration for optimizing your LLM deployment.
Determining optimal batch size for LLM inference
Now that we understand dynamic batching approaches, we need to establish how to select the ideal batch size for your specific workload and hardware configuration. This critical decision impacts both performance and cost-effectiveness.
Finding the right batch size for LLM inference involves balancing throughput, latency, and hardware constraints. This section explores the mathematical framework and key factors for batch size optimization.
Memory-bound vs compute-bound operations
LLM inference is primarily memory-bound rather than compute-bound. The process of loading model parameters from GPU memory consumes more time than the actual computations. This creates a critical ratio between:
- 1Time needed to transfer weights between memory and compute units
- 2Time required for computational operations
When these times are equal, you can increase batch size without performance penalty. Beyond this point, bottlenecks emerge in either memory transfer or computation.
Key factors influencing optimal batch size
Several variables affect the ideal batch size for your deployment:
For instance, a Llama2-70B model in FP16 precision with batch size 1 has a KV cache of approximately 2GB. This grows proportionally with batch size.
Identifying performance plateaus
Research shows clear performance patterns across batch sizes:
Benchmarks with Llama2-70B demonstrate that throughput increases significantly until batch size 64, after which gains plateau while latency continues to rise.
Hardware-specific considerations
Different GPU configurations require different approaches:
- Single A10 GPU: Out-of-memory errors occur beyond batch size 16
- Multiple A100s: Can handle larger batches (up to 128) efficiently
- H100s: Offer 36% lower latency for batch size 1 and 52% lower for batch size 16 compared to A100s
For multi-GPU setups, smaller batch sizes per GPU (around 16) often provide optimal performance.
Practical calculation methodology
To determine maximum batch size for your deployment:
- 1Calculate available GPU memory: Total GPU memory minus model weights
- 2Determine KV cache size per token using the formula:
KV cache size = 2 num_layers hidden_size * precision
- 1Divide available memory by KV cache size to find maximum tokens
- 2Calculate maximum batch size based on expected sequence lengths
This methodical approach ensures you select a batch size that maximizes throughput while operating within your hardware constraints. With your optimal batch size determined, we can now explore techniques to optimize memory management for even greater efficiency.
Memory management techniques for efficient LLM inference
Beyond selecting the right batch size, effective memory management is crucial for maximizing LLM inference performance. Let's examine advanced techniques that can dramatically reduce memory requirements while maintaining or enhancing throughput.
KV cache optimization
LLMs face significant memory constraints during inference, with the Key-Value (KV) cache being a primary bottleneck. Efficient KV cache management employs three key strategies:
When combined, these techniques yield dramatic improvements. For instance, pairing FlashAttention with efficient KV cache management has shown up to a 20x reduction in memory usage for long sequences, all while maintaining speed.
Quantization approaches
Quantization significantly reduces the memory footprint of LLMs during inference. By converting model weights from higher precision (32-bit or 16-bit) to lower precision formats like INT8 or INT4, quantization enables faster inference with minimal accuracy loss.
Memory reduction comparison:
- FP16: 2 bytes per weight
- INT8: 1 byte per weight (50% reduction)
- INT4: 0.5 byte per weight (75% reduction)
The technique is especially effective for weight-memory bound operations. Converting from FP16 (2 bytes) to INT8 (1 byte) or INT4 (0.5 byte) reduces the amount of data transferred during inference, directly accelerating token generation speed.
FlashAttention for memory complexity reduction
FlashAttention revolutionizes attention mechanism efficiency by optimizing memory access patterns. This IO-aware algorithm reduces memory complexity from O(n²) to O(n) by employing tiling techniques that compute small portions of the final matrix at once.
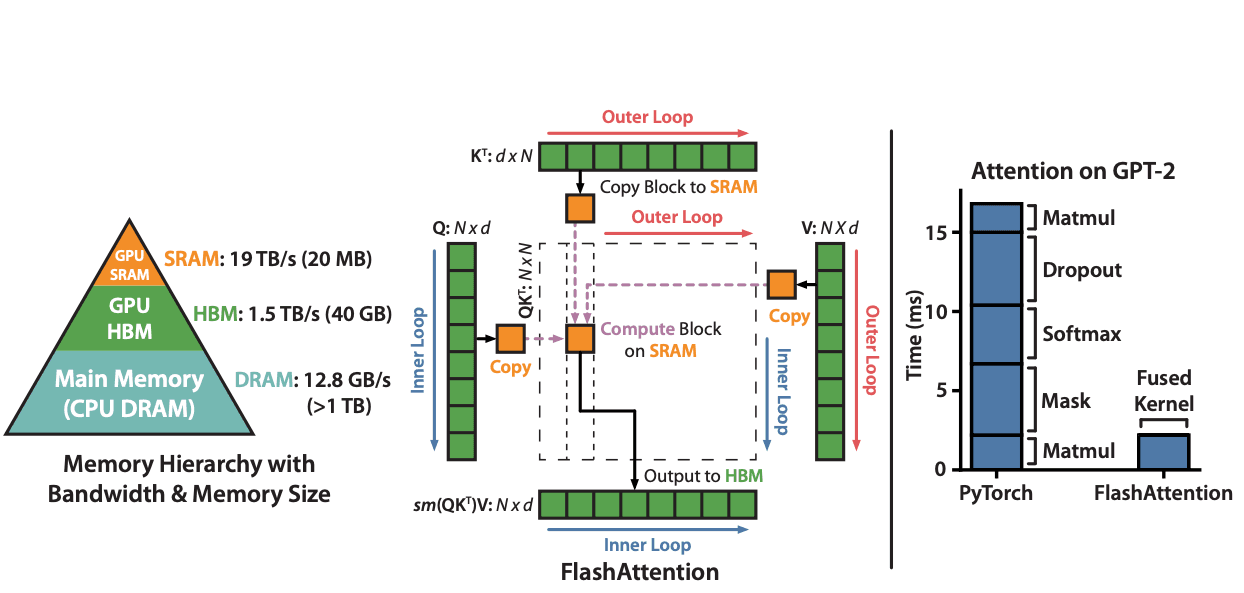
FlashAttention Memory Optimization. Comparison of memory access patterns in traditional attention vs. FlashAttention, showing how tiling and SRAM utilization reduce memory transfers and computation time for LLM inference.
Source: FlashAttention: Fast and Memory-Efficient Exact Attention with IO-Awareness
Instead of performing computation on the entire matrix in steps, FlashAttention fully processes and outputs smaller sections, minimizing intermediate data movement. This approach significantly improves throughput for long sequences while reducing memory overhead.
LoRA adapters for multi-task deployment
Low-Rank Adaptation (LoRA) enables efficient multi-task deployment in memory-constrained environments. Rather than fine-tuning all model parameters, LoRA adds small trainable rank decomposition matrices to selected layers, reducing the parameter count significantly.
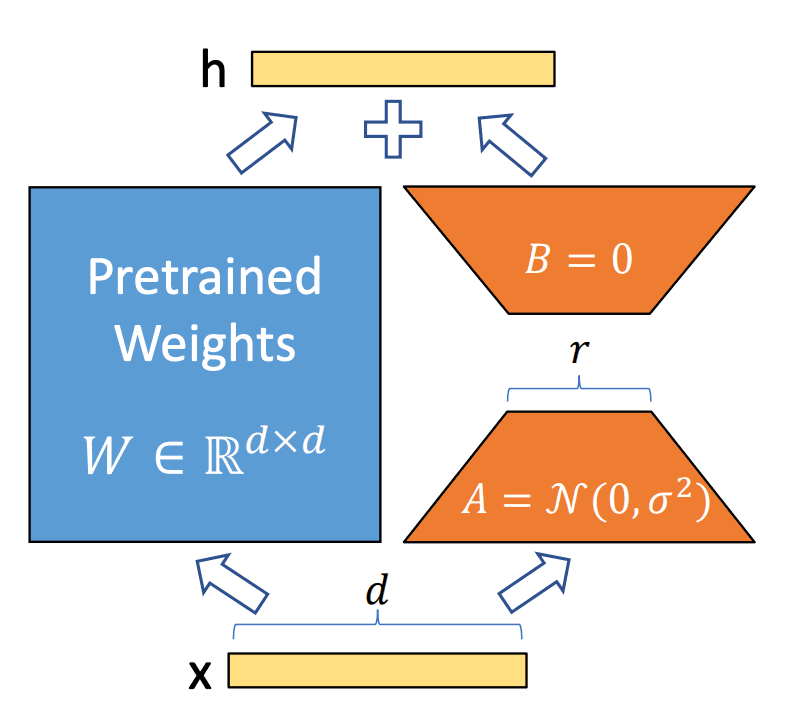
LoRA Architecture Diagram. Visualization of LoRA's parameter-efficient fine-tuning approach, showing how low-rank decomposition matrices (A, B) are added to the original weights (W) to create task-specific adaptations with minimal memory overhead.
Source: LoRA: Low-Rank Adaptation of Large Language Models
This approach is particularly valuable for scenarios requiring multiple specialized models. A single base model can be combined with various compact LoRA adapters (typically under 0.5GB each), enabling efficient switching between specialized tasks without loading entirely separate models.
Performance impact metrics
Memory optimization techniques deliver substantial benefits across multiple dimensions:
A balanced implementation combining quantization, efficient KV cache management, and parallelism techniques provides the best results, especially when tailored to specific hardware configurations. With these memory optimizations in place, we can now address another critical aspect of batch inference: handling inputs of varying lengths.
Variable-length input processing in batch inference
Having optimized memory management, we now tackle another significant challenge in batch inference: efficiently handling inputs of varying lengths. This capability is crucial for real-world applications where prompts naturally differ in size.
Technical challenges
Handling variable-length sequences during batch processing presents significant obstacles for LLM inference. When processing batches, traditional systems pre-allocate fixed-size memory blocks for each sequence's maximum potential length. This leads to substantial memory wastage, often up to 60% of GPU resources, as most sequences won't consume their entire allocated context window.
The computationally intensive attention mechanism poses additional difficulties, as its memory requirements grow quadratically with context length. This makes efficient processing of heterogeneous inputs particularly challenging.
Optimization strategies
Several approaches can mitigate these challenges:
- 1
PagedAttention
Revolutionizes memory allocation by storing KV cache data in non-contiguous memory blocks, enabling dynamic scaling based on actual sequence lengths rather than maximum potential lengths. - 2
Padding strategies
Require careful consideration. While zero-padding ensures uniform sequence length for batch processing, it creates computational inefficiency. Implementing variable-length processing allows the model to focus computational resources only on actual content. - 3
Chunking
Breaking longer sequences into manageable segments can improve memory utilization, especially for workloads with widely varying input lengths.
Implementation architecture
PagedAttention's implementation uses a non-contiguous memory allocation system similar to virtual memory in operating systems. This approach separates the logical view of the KV cache from its physical storage, using a dynamic mapping table to track memory blocks.
Each sequence's KV cache is partitioned into fixed-size blocks that can be stored non-contiguously, with new blocks allocated only as needed. This allows sequences to grow dynamically without pre-allocating space for maximum potential length.
Memory is managed through block tables that efficiently track which physical memory blocks correspond to which parts of different sequences.
Efficiency metrics
With PagedAttention and similar optimizations:
- Memory waste reduced from 60% to less than 4%
- Higher throughput through more efficient memory usage enabling larger batch sizes
- Significant economic impact, making batch inference cheaper and more accessible for production deployments
Code implementation patterns
Effective implementation requires careful token management and memory allocation strategies. For example:
Monitoring systems should track metrics like:
- Memory utilization
- Batch occupancy
- Request latency distribution
These measurements ensure optimization effectiveness in production environments. These variable-length processing capabilities complete our comprehensive approach to batch inference optimization.
Conclusion
Batch inference represents one of the most powerful levers for optimizing LLM-powered products. By grouping multiple requests for simultaneous processing, teams can achieve dramatically better economics - up to 80% cost reduction - while maintaining acceptable performance characteristics.
Key technical takeaways:
- Understanding the memory-bound nature of LLM operations is fundamental
- Continuous batching with techniques like PagedAttention addresses this constraint
- Memory waste reduction from 60% to less than 4% enables higher throughput
- Batch sizes between 32-64 offer optimal balance for most production systems
For product teams:
- These optimizations directly impact what features become economically viable
- Features requiring continuous processing of large document volumes or frequent background LLM calls may only become feasible with proper batch processing
- Consider batch inference as a core architectural pattern rather than an afterthought
For engineers implementing these systems:
- Focus on monitoring memory utilization metrics alongside traditional performance indicators
- The relationship between batch size, latency, and throughput varies significantly across hardware configurations and model sizes
- Systematic benchmarking is essential for optimal tuning
For startup leadership:
- These optimizations directly impact unit economics and infrastructure costs
- Well-implemented batch inference can be the difference between a profitable AI feature and an unsustainable one, particularly as usage scales