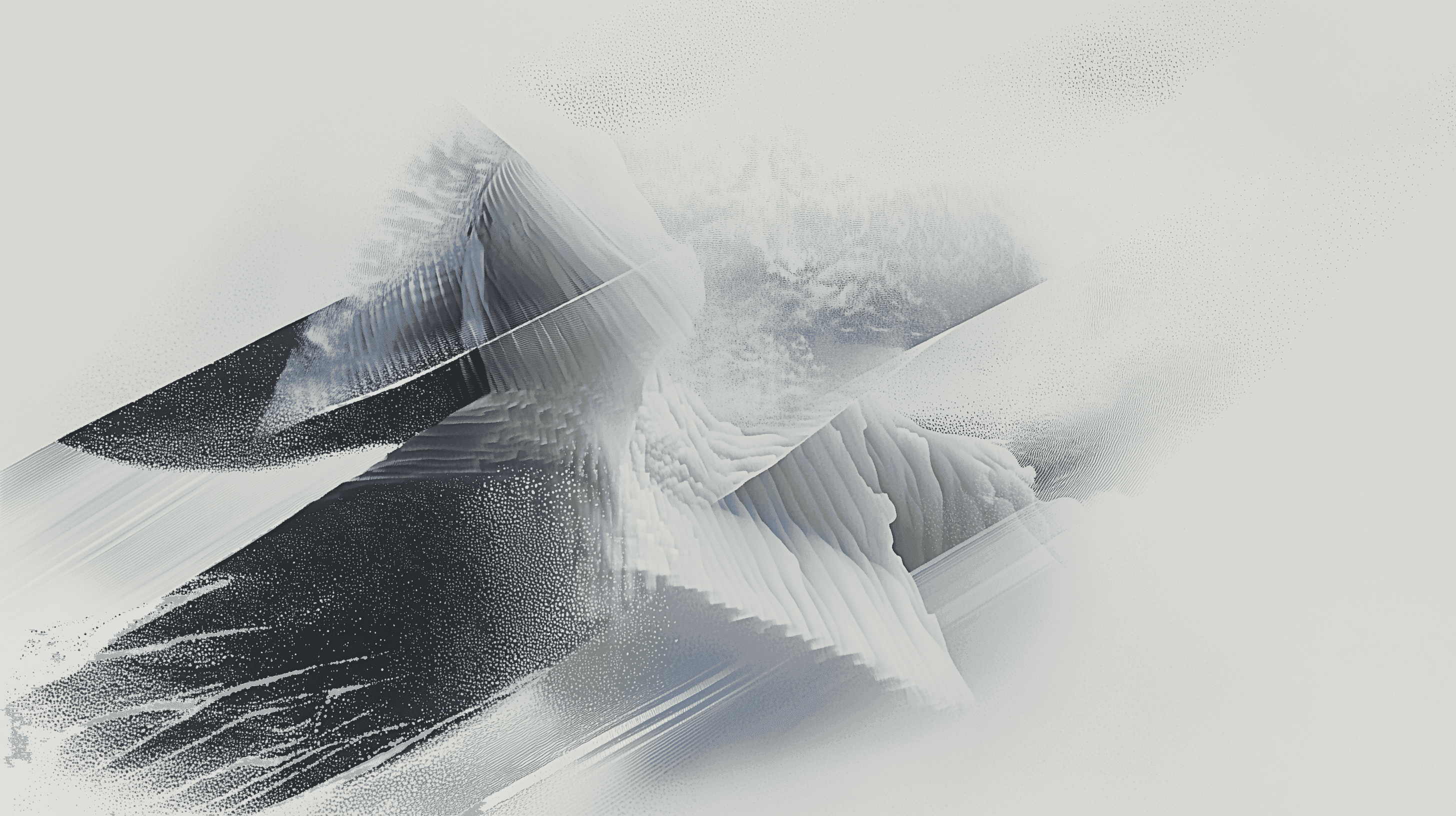
Chain-of-thought (CoT) reasoning is reshaping the development of LLMs by significantly enhancing their logical reasoning and decision-making capabilities. CoT reasoning has fundamentally transformed how Large Language Models (LLMs) process and generate structured reasoning. This blog delves into the technical foundations of CoT, its role in improving AI's logical consistency, and how it's shaping the next wave of LLM development. This technique represents a pivotal shift in how AI systems tackle complex tasks. Rather than producing an answer in one leap, CoT compels an LLM to generate intermediate logical steps—akin to a human meticulously laying out their reasoning.
In this article, I will:
- 1Explain the formal definition of Chain-of-Thought reasoning.
- 2Illustrate the timeline of its development and key milestones.
- 3Delve into the core technical insights, including mathematics and probabilistic models.
- 4Highlight real-world applications and reflect on ongoing challenges.
The overarching goal is to provide a structured, in-depth account of how CoT has evolved from an early-stage research curiosity to a robust, widely adopted method for improving AI reasoning.
What is Chain-of-Thought (CoT) reasoning?
CoTreasoning is a prompting method where the model “thinks out loud,” providing step-by-step breakdowns of its logic. Doing so exposes how each inference step connects to the previous one.
Key points:
- 1
Structured Reasoning
Instead of jumping to the final answer, the model generates successive intermediate states. This approach makes it easier to verify and interpret the AI’s reasoning. - 2
Inspired by Human Cognition
Humans naturally unravel complex questions by parsing them into smaller sub-problems. CoT mimics this, resulting in higher accuracy and improved problem-solving depth. - 3
Early Evidence
Wei et al. (2022) showed that by simply prompting an LLM with "Let’s break this down step by step," the model’s math and logic puzzles performance could increase dramatically.
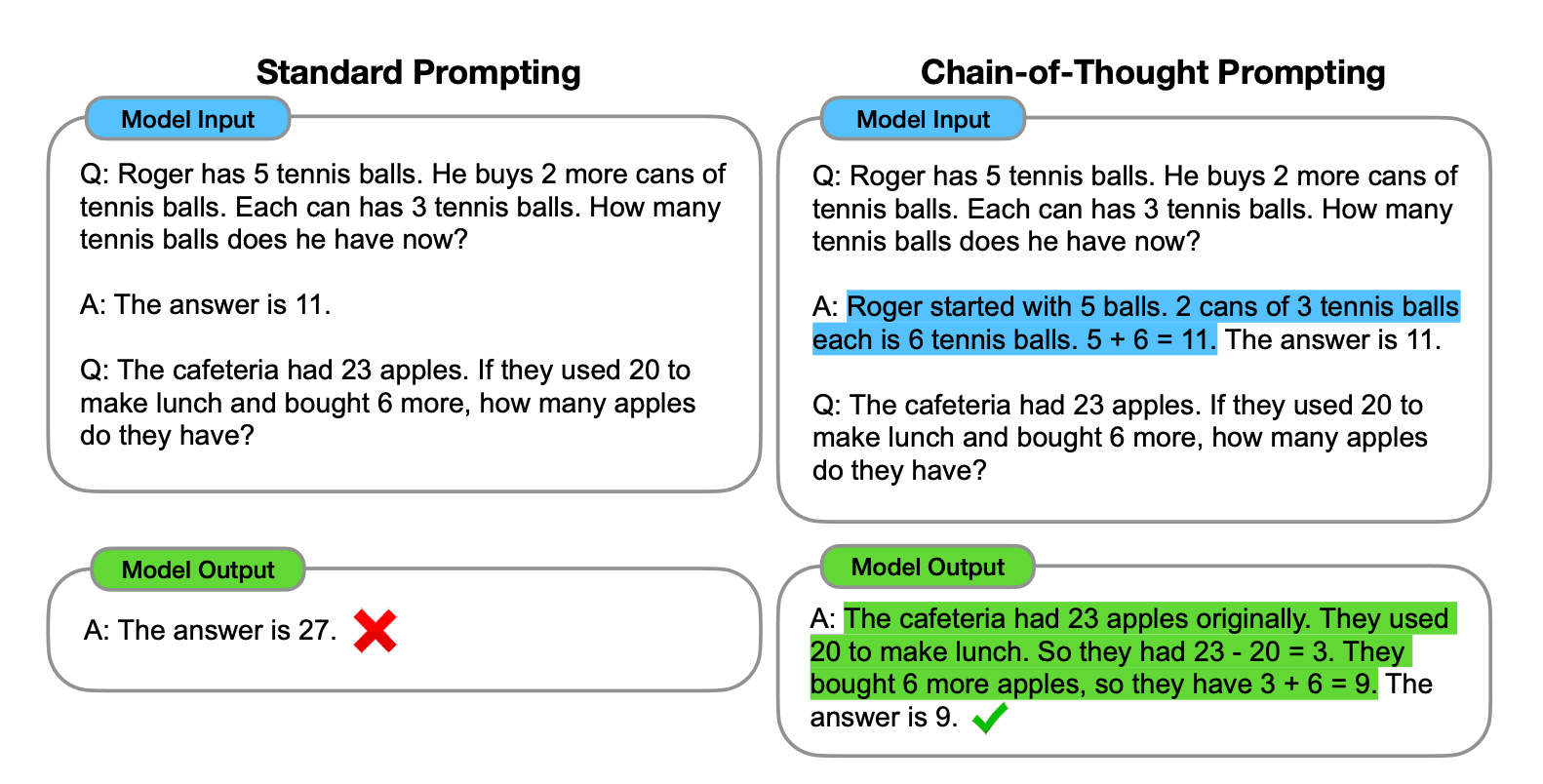
A comparison between standard prompting and chain-of-thought prompting | Source: Chain-of-Thought Prompting Elicits Reasoning in Large Language Models
Timeline: The evolution of Chain-of-Thought
Understanding the historical arc of CoT helps clarify why it’s become so central to AI agent development.
Training with Scaled Reinforcement Learning
Almost all the reasoning models DeepSeek R1, Grok 3, OpenAI o1, and o3 were trained using scaled reinforcement learning, which enables models to refine their reasoning processes through iterative feedback.
This training approach allows the models to explore various strategies, recognize errors, and adjust their internal CoT accordingly. By focusing on reinforcement learning, these companies aim to surpass the limitations of supervised learning, which often relies heavily on human-annotated data and may not scale effectively for complex reasoning tasks.
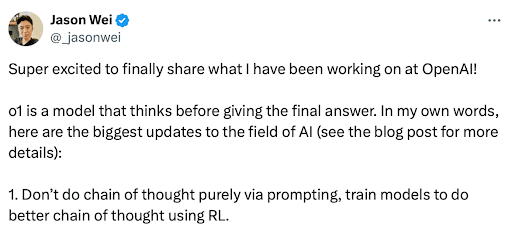
Source: Jason Wei on X
Essentially, pattern recognition.
Benefits of This Training Method
The application of scaled reinforcement learning in training o1 and o3 models offers several advantages:
- 1
Enhanced Problem-Solving
Models can autonomously develop and refine strategies for complex tasks, improving performance in areas like mathematics and coding. - 2
Reduced Dependency on Human Data
By learning from iterative feedback rather than solely relying on human-annotated data, models can achieve superhuman performance levels without being constrained by the quality or quantity of human inputs. - 3
Improved Safety and Alignment
The deliberative alignment mechanism in o3 ensures that the model’s outputs are not only accurate but also align with safety protocols, making AI interactions more reliable and trustworthy.
These developments underscore a significant trend: leading AI companies actively incorporate CoT reasoning into their models to enhance logical processing and decision-making capabilities. This shift improves performance and makes AI outputs more transparent and interpretable, aligning with the growing demand for explainable AI solutions.
Core technical insights
While CoT is often described as a “prompting technique,” there is real mathematical depth that underpins how it works. At the highest level, you can think of each reasoning step as a state in a Markov Decision Process (MDP).
Markov Decision Process (MDP) formulation
- MDP Overview: In an MDP, each new state depends on the previous states, capturing the process of sequential reasoning.
- Probabilistic Model Formally, we define:
where x is the initial query (e.g., a math problem), and st are the stepwise partial solutions. The probability of each state hinges on what came before.
- Interpretation: By structuring the solution path in smaller sub-states, the LLM is effectively searching through a space of possible steps, guided by its attention mechanism.
Attention and logical consistency
- Multi-Head attention: CoT harnesses the transformer’s built-in capacity for focusing on different parts of the sequence at once. This ensures every step is consistent with preceding steps.
- Templating: Models like GPT-4 apply templates such as "Step 1 ... Step 2 ... Answer" to impose a formal structure. This fosters clarity, reduces confusion, and can enhance interpretability.
Tree-of-Thoughts (ToT)
While CoT is typically linear, ToT generalizes the approach:
- Branching: Instead of a single chain, the model branches into multiple potential sequences (DFS or BFS). It explores different reasoning paths and settles on the most promising.
- Example: For a challenging puzzle, one chain might become stuck, while another chain eventually leads to the correct solution.
Multi-Agent CoT
- Collaborative Reasoning: Multiple AI agents can simultaneously generate chain-of-thought solutions and critique each other’s logic.
- Iterative Refinement: This team-based approach can dramatically improve accuracy (e.g., achieving around 89% on the MATH dataset in some studies).
Real-world applications
Chain-of-Thought has moved from research labs into practical AI tools. Below are a few notable domains where CoT has proven invaluable.
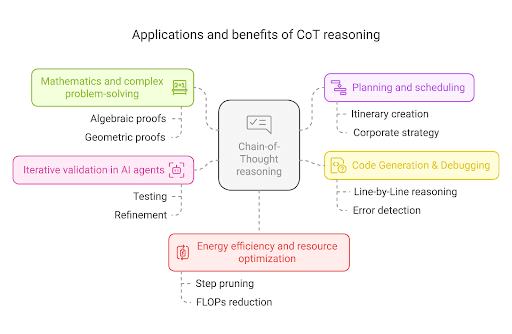
Challenges and Current Limitations
No technique is without flaws, and CoT is still evolving. Here are some pain points.
Knowledge reliance
- 1
Challenge
CoT depends on robust pre trained knowledge. If the model is unfamiliar with the domain, stepwise reasoning may crumble. - 2
Example
A model might produce a logical series of steps about quantum computing but rely on incomplete or incorrect training data.
Hallucinations and fabricated steps
- 1
Challenge
Some steps can be entirely made up, leading to artificially confident but false conclusions. - 2
Mitigation
Lowering the temperature (0.3–0.7) and employing external fact-checking can reduce these hallucinations.
Mechanistic interpretability
- 1
Challenge
Even if the CoT is spelled out, we still do not always understand the model’s deeper transformations. Many steps might be heuristic shortcuts. - 2
Research focus
Ongoing projects aim to decode how exactly transformers weigh tokens at each step, bridging the gap between “explanation” and actual cognition.
Ethical and bias considerations
- 1
Challenge
Stepwise reasoning can inadvertently preserve or even amplify biases in the training corpus. - 2
Future solutions
Tools that evaluate each step for bias or unethical reasoning patterns before finalizing the output.
Scalability and efficiency
- 1
Challenge
Generating a fully transparent CoT can require significant computational overhead for huge tasks. - 2
Potential fix
Selective chain-of-thought expansions or truncated reasoning steps to balance clarity and performance.
The Future of CoT
CoT is not a static technique. As LLMs, hardware, and research paradigms advance, so too will CoT.
- 1
Emerging Techniques
Novel spin-offs like Tree-of-Thoughts are already merging CoT with advanced search strategies to explore solution paths systematically. - 2
Agent Evolution
We anticipate AI agents that use CoT to dynamically self-correct, consult external APIs, or recruit other specialized agents when encountering domain-specific queries. - 3
GPT-5 and Beyond
The next wave of large-scale models may incorporate CoT at a foundational level rather than as an optional prompting trick. - 4
Societal Impact
Widespread CoT usage could help produce more transparent and trustworthy AI, though bias concerns and interpretability challenges remain a top priority.
Conclusion
CoT reasoning has significantly reshaped how we approach problem-solving in LLMs. Starting from its initial conception in 2022 (Wei et al.), CoT has evolved into an umbrella of methods—encompassing multi-agent collaboration, branching search (ToT), and real-time verification.
Key takeaways:
- 1
Structured reasoning
CoT forces an LLM to lay out its thought process, improving interpretability. - 2
Proven benchmarks
Substantial leaps in accuracy (e.g., ~34% to ~74% in math tasks) underscore its practical benefits. - 3
Industry adoption
CoT is transforming AI agents across multiple verticals, from code debugging to corporate strategy.
Ongoing questions:
- 1
Interpretability
How do we distinguish authentic reasoning from memorized heuristics? - 2
Ethical concerns
Are we inadvertently reinforcing biases? How can we add checks at each step? - 3
Scalability
Will more advanced forms of CoT keep pace with the rising complexity of tasks?
Broader vision
CoT’s biggest promise is to bring together deep learning and human-like thinking. By compelling models to produce a rationale for each step, we may inch closer to truly explainable AI. Meanwhile, the role of human oversight—especially in identifying bias or hallucinations—remains vital.
CoT is one of the most transformative techniques to emerge in recent AI history. Its structured logic, potential for transparency, and capacity for handling complex tasks herald a new era of AI solutions that can reason—and occasionally even reflect—much like we do.