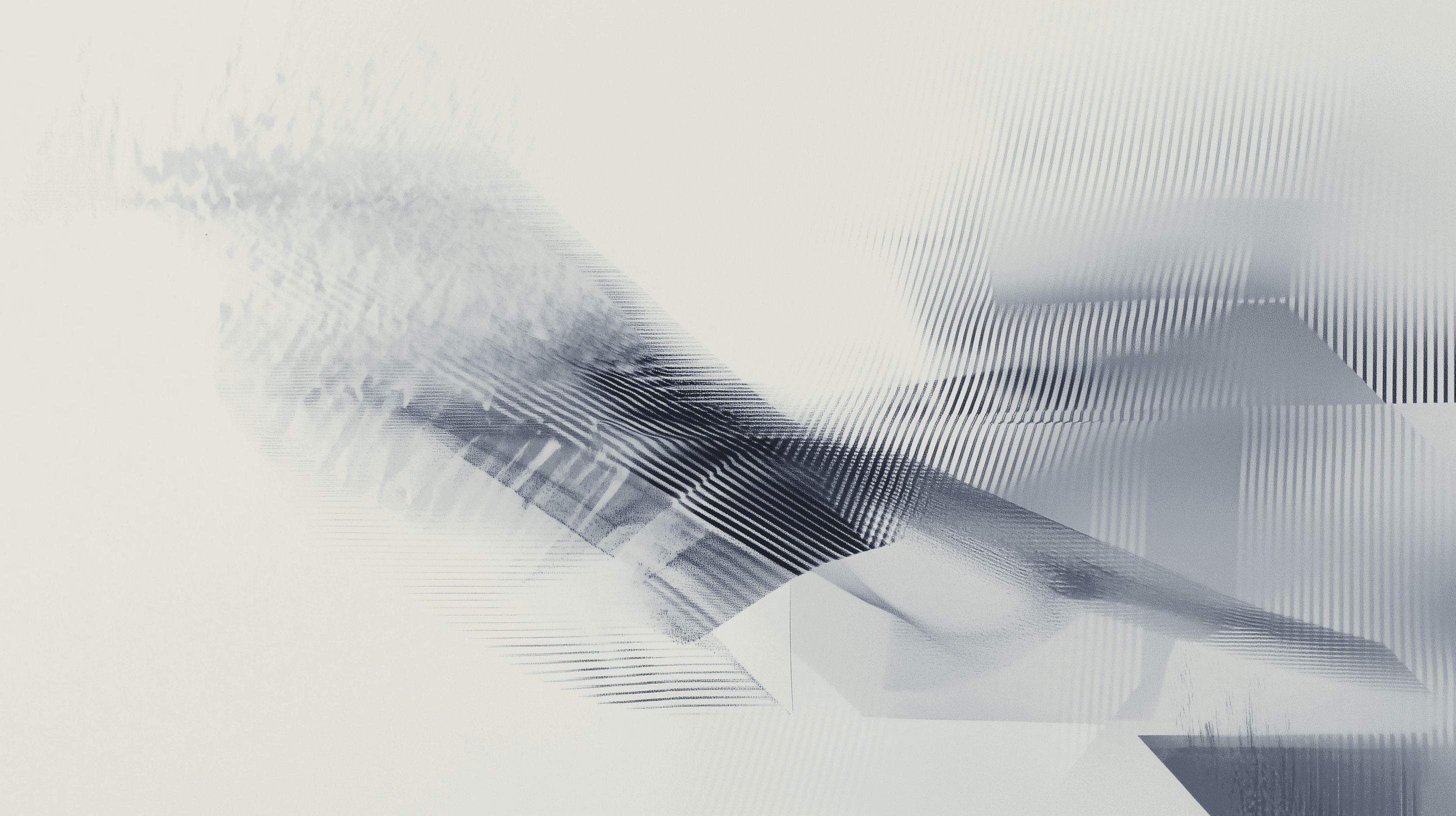
The distinction between open-source and open-weight LLMs represents a crucial decision point for AI teams building modern applications. While these terms are often used interchangeably, they represent fundamentally different approaches to model transparency, customization capabilities, and legal frameworks that directly impact implementation strategy. Your choice between these model types will significantly influence development flexibility, compliance requirements, and long-term operational costs.
This comprehensive analysis examines the technical and legal distinctions separating these model categories. We'll explore how open-source models provide complete access to architecture, training methodology, and weights under permissive licenses, while open-weight alternatives share only parameter weights with more restrictive terms. These differences create cascading effects on everything from bias detection to architectural customization.
Understanding these distinctions enables more strategic AI implementation decisions. Teams gain clarity on the performance trade-offs, resource requirements, and risk profiles associated with each approach, allowing for more informed architectural choices aligned with business requirements and technical capabilities.
Key Areas Covered:
- 1Core definitions and technical distinctions
- 2Licensing frameworks and legal implications
- 3Training methodology transparency considerations
- 4Model architecture access and customization capabilities
- 5Strategic implementation decision framework
Core definitions and technical distinctions between open-source and open-weight models
As we explore this critical area, it's essential to understand exactly what these terms mean and how they differ in practical implementation.
What are open-source AI models?
Open-source models provide complete access to the entire model architecture, training methodology, and weights. This comprehensive access includes the model code, architecture design, training scripts, and parameter weights under licenses like MIT or Apache. The transparency allows users to fully examine how the model was created and modify it as needed. Open-source models embody true transparency, enabling researchers to reproduce results, audit for security vulnerabilities, and customize the model for specific applications.
Technical components of open-source models include:
- Model architecture code defining the neural network structure
- Training scripts and methodologies
- Parameter weights learned during training
- Documentation of training datasets
- Licensing that permits modification and redistribution
This level of openness provides unparalleled flexibility for researchers and developers seeking to understand or modify AI systems.
What are Open-weight models?
Open-weight models represent a more limited approach to model sharing. These models release only the trained parameter weights while restricting access to training methodologies and code. This distinction is significant for several technical reasons:
- Only the model weights are accessible, not the training pipeline
- Training code, data preparation methods, and optimization techniques remain proprietary
- More restrictive licenses often limit commercial use or modification
- The reproducibility of model training becomes technically challenging
This approach has gained popularity as organizations seek to balance transparency with intellectual property protection.
Comparative analysis of technical components
When examining the technical components available between these two model types, several key distinctions emerge:
These differences significantly impact how models can be used and modified in practice.
Technical implementation differences
The distinction between model weights and complete model architecture significantly impacts technical implementation. Model weights alone represent learned parameters but lack the framework that defines how these parameters interact. Without the architecture code, developers must reverse-engineer or make assumptions about:
- Layer connections and activation functions
- Tokenization methods and vocabulary handling
- Input preprocessing requirements
- Output postprocessing techniques
This missing technical information creates friction for developers attempting to implement or modify open-weight models.
Impact on technical capabilities
These differences directly affect what users can accomplish with each model type:
Open-source advantages:
- Complete reproducibility enables validation of model behavior
- Architecture modifications allow for fundamental model improvements
- Custom training enables domain-specific adaptations
- Security auditing can examine the entire model pipeline
Open-weight limitations:
- Restricted to using the model as provided
- Limited ability to address fundamental model biases
- Constrained customization options
- Reduced transparency for security auditing
The tension between these approaches reflects the balance between openness and intellectual property protection, particularly as models demonstrate increasingly sophisticated reasoning capabilities that represent competitive advantages in the AI landscape.
These technical distinctions form the foundation for understanding broader implications in licensing, implementation, and strategic decision-making that we'll explore in subsequent sections.
Licensing frameworks and legal implications for AI implementation
Building on our technical understanding, let's examine how licensing structures create distinct legal frameworks that govern implementation options for these model types.
Understanding AI model licensing
The licensing of AI models is a complex landscape with significant implications for deployment and use. Open-source models provide complete access to model code, architecture, training methodology, and weights under licenses like MIT or Apache. These licenses allow for full transparency and modification rights. In contrast, open-weight models release only trained parameter weights while restricting access to training code, typically under more restrictive licenses that may limit commercial or modification rights.
Common licensing structures
Several licensing frameworks govern the use of AI models:
- 1
Apache 2.0
A permissive license allowing commercial use, distribution, modification, and patent use, requiring only preservation of copyright and license notices and documentation of changes. - 2
MIT
An even simpler permissive license with minimal conditions, requiring only preservation of copyright and license notices. - 3
GPL 3.0
A strong copyleft license requiring source code disclosure and licensing of derivative works under the same terms. - 4
Custom licenses
Many organizations implement custom licenses with specific restrictions. Meta's Llama license, for example, requires payment for users with over 700 million monthly active users.
These different licensing frameworks create distinct pathways for implementation, with varying degrees of flexibility and restriction.
Commercial usage rights and restrictions
Licensing terms significantly impact how AI models can be used in commercial settings. Understanding these nuances is essential for implementation:
- Open-source licenses like Apache 2.0 and MIT generally permit unrestricted commercial use.
- Some open-weight models impose limitations on commercial applications or require payment beyond certain usage thresholds.
- Custom licenses often include specific commercial use restrictions, particularly for large-scale deployments.
- Many modern licenses explicitly prohibit using model outputs to train competing AI systems.
These commercial considerations can dramatically impact the total cost of ownership and flexibility for business applications.
Legal implications for derivative works
The creation of derivative works through fine-tuning presents unique legal challenges:
- License terms may determine whether fine-tuned versions maintain the original license or can adopt new terms.
- Some licenses require derivative works to maintain the same open-source status as the original model.
- Modifications to open-weight models may face additional restrictions compared to fully open-source alternatives.
- Legal risks increase when combining models with different licensing frameworks or when fine-tuning without proper documentation.
This complexity around derivative works becomes particularly important for organizations seeking to create customized versions of existing models.
Risk assessment framework for compliance
To mitigate legal risks when implementing AI models, consider this assessment framework:
- 1
Documentation review
Maintain comprehensive records of model origins, licenses, and modifications. - 2
Usage analysis
Evaluate your implementation against specific license restrictions, particularly regarding scale and commercial applications. - 3
Modification tracking
Document all fine-tuning efforts and their relationship to original models. - 4
Regular audits
Conduct periodic reviews of AI implementations to ensure ongoing compliance with licensing terms. - 5
Legal consultation
Seek specialized legal advice when implementing models with complex licensing structures or for large-scale deployments.
This systematic approach helps organizations navigate the complex licensing landscape while minimizing legal exposure in AI implementation.
The licensing considerations discussed here demonstrate how the choice between open-source and open-weight models extends beyond technical factors into legal and compliance territories that organizations must carefully navigate.
Training methodology and data transparency considerations
Now that we understand the technical and legal distinctions, let's examine how these model types differ in transparency around their training processes - a critical factor for responsible AI implementation.
Understanding training data opacity
Training data transparency varies significantly across open-weight models. Many providers release model weights without disclosing their training methodologies or datasets. This opacity creates challenges for researchers and organizations seeking to evaluate model reliability. Without insight into training data composition, users cannot fully assess potential biases or determine if models were exposed to sensitive or problematic content.
When training methodologies remain hidden, organizations face increased governance challenges. Transparency gaps make it difficult to verify compliance with regulatory requirements or ethical guidelines. This issue is particularly significant in regulated industries like finance and healthcare.
Models with limited training transparency also present unique reproducibility hurdles. Without access to training methodologies, researchers cannot verify claims or replicate results independently.
Detecting bias with limited information
Even with restricted training transparency, several approaches can help detect and mitigate biases:
- Benchmark testing across diverse demographic groups
- Adversarial testing with edge cases
- Implementation of bias monitoring frameworks
- Regular auditing of model outputs
- Use of fairness metrics such as demographic parity
Studies indicate that implementing these techniques can increase model performance by up to 20% in underrepresented groups. Cross-validation with varied datasets helps identify performance discrepancies that might drop from 90% accuracy on one dataset to 60% on another due to underlying biases.
Impact on reproducibility
Training methodology opacity significantly impacts research reproducibility. Approximately 50% of researchers report difficulties reproducing results due to insufficient documentation of parameter settings, data splits, and evaluation metrics. This reproducibility crisis undermines scientific progress and makes building upon existing work challenging.
When training processes lack transparency, organizations must implement additional validation steps. These may include:
- Establishing robust baseline comparisons against multiple methods
- Thorough documentation of evaluation procedures
- External validation from third-party specialists
- Continuous feedback loops to refine assessment processes
Practical implementation challenges
For startups implementing models with limited training transparency, several practical considerations emerge:
- 1Data security protocols must be enhanced to protect against potential vulnerabilities inherited from base models.
- 2Risk assessment frameworks should account for the unknown elements of model training.
- 3Monitoring systems need to track model performance over time to detect drift.
- 4Clearly defined accountability structures become essential.
A single organization may not have resources to comprehensively address these challenges, making collaborative approaches and industry standards increasingly important.
Balancing transparency with pragmatism
While complete training transparency remains ideal, organizations must often make practical compromises. Implementing documentation frameworks that detail how models are used, even if their training remains opaque, can enhance accountability. Regular audits of model outputs provide an alternative approach to understanding model behavior when training data remains inaccessible.
For critical applications, consider employing model ensembles that leverage multiple open-weight models with different architectures. This diversity can help mitigate the risks associated with any single model's unknown training methodology.
Remember that transparency exists on a spectrum. Even with limited training information, ethical implementation practices and robust monitoring can significantly improve outcomes.
These transparency considerations highlight the additional governance challenges that emerge when implementing models with limited visibility into their development process - a factor that must be weighed carefully in the decision between open-source and open-weight approaches.
Training methodology and data transparency considerations
As we continue our exploration of transparency issues, let's examine the practical implications for organizations implementing these different model types.
Understanding training data opacity
Training data transparency varies significantly across open-weight models. Many providers release model weights without disclosing their training methodologies or datasets. This opacity creates challenges for researchers and organizations seeking to evaluate model reliability. Without insight into training data composition, users cannot fully assess potential biases or determine if models were exposed to sensitive or problematic content.
When training methodologies remain hidden, organizations face increased governance challenges. Transparency gaps make it difficult to verify compliance with regulatory requirements or ethical guidelines. This issue is particularly significant in regulated industries like finance and healthcare.
Models with limited training transparency also present unique reproducibility hurdles. Without access to training methodologies, researchers cannot verify claims or replicate results independently.
Detecting bias with limited information
Even with restricted training transparency, several approaches can help detect and mitigate biases:
- Benchmark testing across diverse demographic groups
- Adversarial testing with edge cases
- Implementation of bias monitoring frameworks
- Regular auditing of model outputs
- Use of fairness metrics such as demographic parity
Studies indicate that implementing these techniques can increase model performance by up to 20% in underrepresented groups. Cross-validation with varied datasets helps identify performance discrepancies that might drop from 90% accuracy on one dataset to 60% on another due to underlying biases.
Impact on reproducibility
Training methodology opacity significantly impacts research reproducibility. Approximately 50% of researchers report difficulties reproducing results due to insufficient documentation of parameter settings, data splits, and evaluation metrics. This reproducibility crisis undermines scientific progress and makes building upon existing work challenging.
When training processes lack transparency, organizations must implement additional validation steps. These may include:
- Establishing robust baseline comparisons against multiple methods
- Thorough documentation of evaluation procedures
- External validation from third-party specialists
- Continuous feedback loops to refine assessment processes
Practical implementation challenges
For startups implementing models with limited training transparency, several practical considerations emerge:
- 1Data security protocols must be enhanced to protect against potential vulnerabilities inherited from base models.
- 2Risk assessment frameworks should account for the unknown elements of model training.
- 3Monitoring systems need to track model performance over time to detect drift.
- 4Clearly defined accountability structures become essential.
A single organization may not have resources to comprehensively address these challenges, making collaborative approaches and industry standards increasingly important.
Balancing transparency with pragmatism
While complete training transparency remains ideal, organizations must often make practical compromises. Implementing documentation frameworks that detail how models are used, even if their training remains opaque, can enhance accountability. Regular audits of model outputs provide an alternative approach to understanding model behavior when training data remains inaccessible.
For critical applications, consider employing model ensembles that leverage multiple open-weight models with different architectures. This diversity can help mitigate the risks associated with any single model's unknown training methodology.
Remember that transparency exists on a spectrum. Even with limited training information, ethical implementation practices and robust monitoring can significantly improve outcomes.
Having explored the transparency considerations, we can now turn our attention to how these different approaches affect customization capabilities - another crucial factor in implementation decisions.
Model architecture access and customization capabilities
When implementing AI models, the level of architectural access directly impacts customization options, performance optimization, and deployment flexibility - creating significant differences between open-source and open-weight approaches.
Open-source vs. open-weight approaches
Open-source models provide complete access to the model's code, architecture, training methodology, and weights. These models are typically distributed under permissive licenses like MIT or Apache, allowing full transparency and modification rights. In contrast, open-weight models release only the trained parameter weights while restricting access to training code or methodologies, often under more restrictive licenses that may limit commercial use or modifications.
This distinction has become increasingly important in the current LLM landscape, where advanced reasoning capabilities represent significant competitive advantages for organizations.
Full architectural control benefits
Having complete access to a model's architecture provides several advantages:
- Deeper customization: The ability to modify network structure, attention mechanisms, and core components to suit specific applications
- Enhanced transparency: Better understanding of model behavior and decision-making processes
- Flexible deployment: Freedom to implement the model across various hardware configurations
Organizations with strict compliance regulations or data localization requirements especially benefit from this level of control.
Performance implications
The level of architectural access significantly impacts performance optimization possibilities:
- Weight-only models generally restrict optimization to post-training techniques like quantization and pruning
- Full open-source implementations allow for more fundamental architectural modifications that can yield greater performance improvements
For instance, quantization approaches like GGUF and EXL2 can reduce memory requirements from 32-bit to as low as 2-bit precision, but their effectiveness varies based on the level of architectural access available.
Extension and customization approaches
Several technical approaches exist for extending LLM capabilities based on the level of access:
For weight-only models:
- Prompt engineering and in-context learning
- Retrieval-augmented generation (RAG)
- Basic quantization of provided weights
- API-based deployment with limited customization options
For full open-source models:
- Architecture pruning to eliminate less critical components
- Knowledge distillation to create smaller, specialized models
- Custom attention mechanism implementation
- Hardware-specific optimization at the architectural level
Cost-benefit considerations
When implementing LLMs in production, organizations must weigh several factors:
- Open-source models typically require more technical expertise but offer greater customization potential
- Open-weight models provide easier implementation but may incur higher operational costs due to limited optimization options
- Full architectural access enables more efficient resource utilization but requires significant engineering investment
The ideal approach depends on your specific requirements for control, performance, and resource constraints.
As reasoning capabilities in LLMs continue to advance, the trade-offs between transparency, customization, and ease of implementation will remain a critical consideration for technical teams deploying these systems in production environments.
With an understanding of the technical capabilities, customization options, and performance implications, we can now examine how these factors influence strategic implementation decisions.
Strategic implementation decision framework for technical teams
Drawing together all the aspects we've explored, this section provides a practical framework for making informed decisions between open-source and open-weight approaches.
Open-source vs open-weight models: Key considerations
Choosing between open-source and open-weight models presents distinct trade-offs for technical teams. Open-source models provide complete access to code, architecture, and weights under permissive licenses like MIT or Apache. Open-weight models release only parameter weights with more restrictive usage terms. This distinction has become increasingly important as models demonstrate advanced reasoning capabilities that represent significant competitive advantages.
The decision framework must evaluate several dimensions to identify the optimal approach for your use case.
Resource requirements comparison
Technical expertise
Open-source models require deeper technical knowledge for deployment, customization, and maintenance. Teams must understand model architecture and training methodologies. Open-weight models demand less expertise for basic implementation but offer limited customization options.
Compute resources
Open-weight models typically need less computational power for deployment since they don't support extensive modification. Open-source models may require substantial compute resources for fine-tuning or further training.
Implementation timeline
Open-weight models enable faster deployment through straightforward API integration. Open-source implementation timelines are longer due to customization and infrastructure setup requirements.
Cost analysis across model lifecycle
Initial costs for open-weight models are lower but increase with usage volume. API-based pricing becomes expensive at scale. A single high-traffic application can quickly exceed budgeted costs.
Open-source models involve higher upfront investment in infrastructure and expertise. However, long-term operational costs stabilize regardless of usage growth. This makes open-source more cost-effective for applications with consistent, high-volume usage patterns.
Maintenance costs differ significantly. Open-weight models offload maintenance to providers. Open-source requires ongoing updates, security patching, and optimization by internal teams.
Risk assessment matrix
Business considerations
Open-weight models present risks in vendor dependency, unpredictable pricing changes, and potential service discontinuation. Open-source models mitigate these risks through greater control but require more internal governance.
Data privacy represents another critical factor. Open-weight models typically process data externally, raising concerns for sensitive information. Open-source allows complete data isolation within controlled environments.
Technical considerations
Model performance stability varies between approaches. Open-weight models may change without notice, affecting application reliability. Open-source provides version control and ensures consistent performance.
Security vulnerabilities present different risks. Open-weight models benefit from provider security teams but share vulnerabilities across all users. Open-source allows immediate patching but requires vigilant monitoring.
Implementation pathways
Many organizations benefit from starting with open-weight models for rapid deployment and proof of concept. As technical capabilities mature, a gradual transition to open-source becomes viable.
This migration path should include parallel running periods to compare performance and validate capabilities. Teams must develop expertise in model fine-tuning, infrastructure management, and performance optimization before completing the transition.
For critical applications, maintaining both options provides resilience against service disruptions and leverages the strengths of each approach.
By systematically evaluating these factors, organizations can develop a strategic roadmap that aligns their model implementation approach with their specific requirements, capabilities, and long-term objectives.
Conclusion
The distinction between open-source and open-weight models extends far beyond simple terminology differences. As we've explored, these approaches represent fundamentally different philosophies about AI transparency, customization, and governance that directly impact implementation strategy.
Open-source models provide complete access to architecture, code, and weights—enabling deeper customization, enhanced transparency, and greater deployment flexibility. However, they demand stronger technical expertise and infrastructure investment. Open-weight models offer faster implementation paths with lower initial costs but introduce vendor dependencies, scaling cost concerns, and limitations on architectural optimization.
For product teams, this distinction requires careful roadmap consideration. Initially deploying open-weight models might accelerate time-to-market, while planning for potential migration to open-source as usage scales could optimize long-term costs.
AI engineers should evaluate whether their use cases demand architectural customization or if weight-based optimization suffices. The inability to inspect training methodologies in open-weight models creates additional verification requirements that engineering processes must address.
Strategically, organizations must balance immediate implementation needs against long-term control, cost predictability, and compliance requirements when selecting their approach to LLM integration.
The decision between open-source and open-weight models represents one of the most consequential choices in AI implementation strategy, with far-reaching implications for technical capabilities, operational costs, and governance requirements. By understanding these distinctions, organizations can make more informed decisions aligned with their specific needs and constraints.