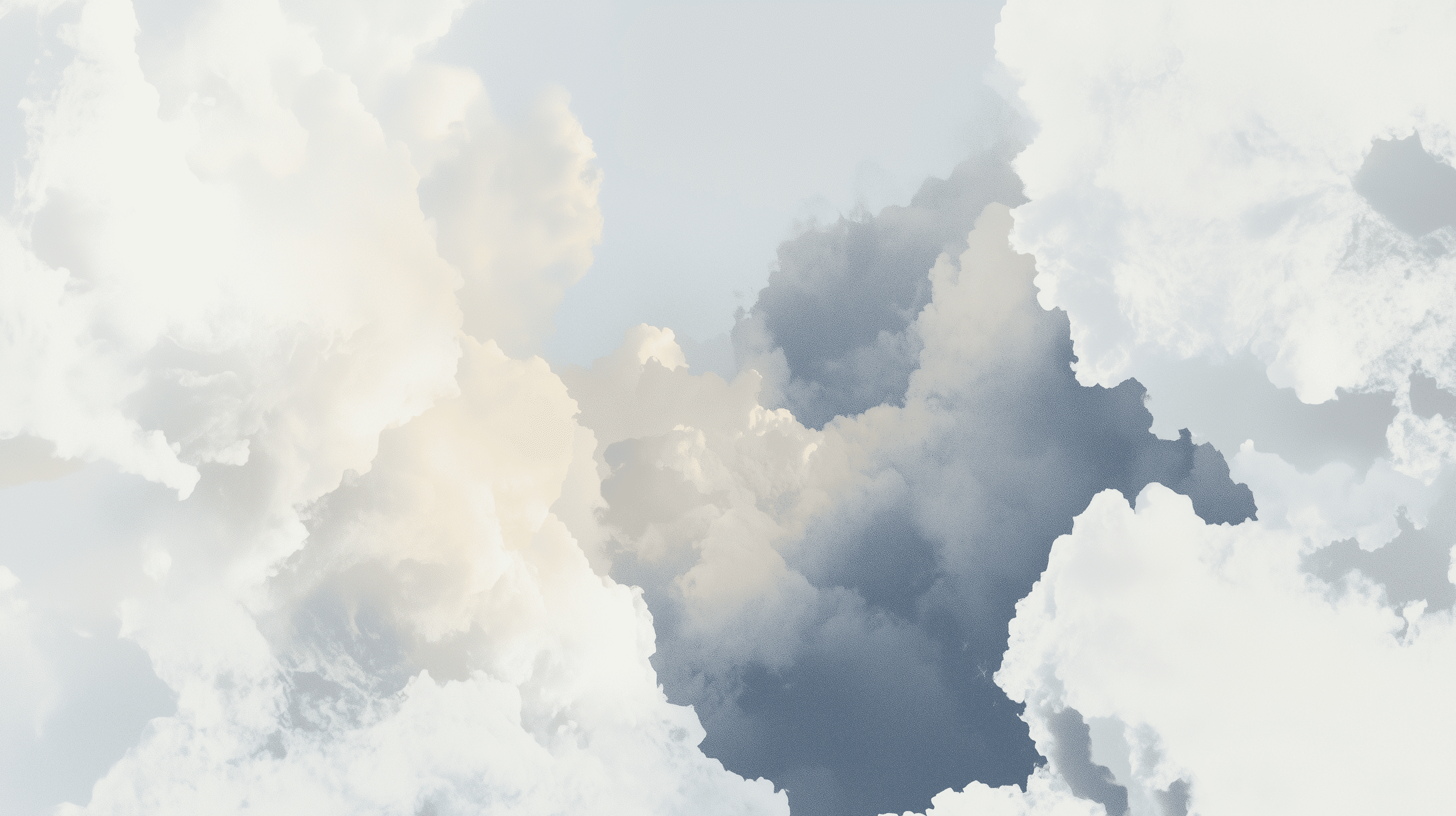
Large language models power modern AI applications, but their remarkable capabilities often come with a critical drawback: opacity. As product teams deploy increasingly sophisticated LLMs, the inability to understand how these systems reach conclusions creates significant business risks. Interpretability—the capacity to understand model behavior and decision processes—has emerged as a crucial factor separating successful AI products from those that fail in the market.
This article explores the technical foundations of LLM interpretability, examining key mechanisms like attention visualization, probing tasks, and layer-wise analysis. We'll contrast interpretability (understanding internal mechanisms) with explainability (justifying specific outputs) and demonstrate how transparency frameworks can be implemented without sacrificing performance.
Transparent AI systems deliver concrete benefits:
- Accelerated debugging cycles
- Stronger user trust
- Simplified regulatory compliance
- Reduced liability
Product teams that master interpretability techniques gain a significant competitive advantage in industries where AI adoption faces scrutiny.
Key Topics:
- 1Defining interpretability and its business impact
- 2Core technical components of transparent LLMs
- 3Regulatory requirements across regions
- 4Measurement frameworks for assessing transparency
- 5Implementation methods including chain-of-thought and RAG
- 6Real-world case studies of success and failure
Let's begin by establishing a clear understanding of what interpretability means in the context of LLMs and why it matters for product development.
Defining interpretability in LLMs: Foundations for product teams
Understanding interpretability vs. explainability
Interpretability in large language models refers to the ability to understand how these models process inputs and generate outputs. This differs from explainability, which focuses on why a model made a particular decision. While interpretability examines the internal mechanisms, explainability provides justifications for specific outputs.
Product success increasingly depends on interpretable AI systems. Users demonstrate higher adoption rates when they understand how decisions are being made. This transparency builds the trust necessary for widespread implementation.
This distinction between interpretability and explainability provides the foundation for developing truly transparent AI systems that users can trust and understand.
Core components of interpretable LLMs
Interpretable AI systems contain several essential elements:
- Mechanisms that provide visibility into model reasoning
- Documentation of decision processes
- Frameworks that quantify confidence levels
Key Techniques for Interpretability:
- 1
Attention mechanisms
By visualizing attention weights, teams can identify which parts of the input influenced particular outputs - 2
Probing tasks
These evaluate a model's understanding of specific linguistic properties and reveal how the model processes different types of information - 3
Layer-wise analysis
Examines how information flows through the model's architecture, helping pinpoint where and how transformations occur during processing
These technical components work together to create LLMs that provide insight into their inner workings, establishing the basis for transparent AI systems.
Regulatory landscape for LLM interpretability
Emerging AI regulations across Europe, North America, and Asia increasingly mandate model transparency. These frameworks require varying degrees of interpretability depending on the application's risk level and potential impact.
Beyond Compliance: Business Advantages
- Faster debugging cycles
- More efficient model improvements
- Improved operational efficiency
- Enhanced product quality
- Accelerated development timelines
Compliance represents just one dimension of the interpretability imperative. Beyond regulatory requirements, interpretable systems provide significant business advantages.
Understanding these regulatory requirements is crucial for product teams as they navigate the complex landscape of AI governance while capitalizing on the business benefits of interpretable systems.
Measurement frameworks for interpretability
Quantifying interpretability presents unique challenges. Several frameworks offer structured approaches to this assessment throughout the product lifecycle.
Implementation requires balancing computational overhead with explanatory power. Some frameworks demand additional processing time but deliver detailed explanations.
These measurement frameworks provide product teams with concrete methods to evaluate and improve the interpretability of their LLM implementations.
Balancing performance with transparency
The relationship between model performance and interpretability often involves tradeoffs. Simpler, more transparent models may sacrifice some capabilities that complex "black box" systems can achieve.
Decision Factors for Balance:
- Specific use case requirements
- Criticality of application
- Cross-functional alignment needs
- Interpretability expertise available
Product teams must determine the appropriate balance for their specific use cases. Critical applications often warrant prioritizing interpretability even at some cost to raw performance.
This balancing act represents one of the central challenges in developing LLM-powered products. Teams need cross-functional alignment and interpretability expertise to navigate these decisions effectively.
Embracing interpretability is not merely a compliance exercise but a strategic investment. Transparent AI systems build stronger user confidence and reduce liability risks, ultimately leading to more successful products in the marketplace.
Having established the foundational concepts of interpretability, let's explore how transparent AI systems directly impact user trust and adoption.
Building user trust through transparent AI
The black box dilemma in AI systems
The increasing adoption of LLMs across industries creates a significant trust challenge. As these models grow more sophisticated, they often function as "black boxes," making their inner workings difficult to understand. This lack of transparency raises concerns, especially in critical applications such as healthcare, finance, and legal sectors, where interpretability is paramount. Trust and accountability are essential in deploying AI systems, and that's where interpretability and explainability become critical.
Critical Sectors Requiring Transparency:
- 1Healthcare
- 2Finance
- 3Legal
- 4Education
- 5Government services
Users deserve to know how decisions affecting them are reached. This transparency ensures AI-driven decisions are free from biases and adhere to ethical principles. Understandable LLMs represent a step toward more responsible AI.
This black box dilemma highlights why interpretability has become such a crucial factor in determining whether users will accept or reject AI-powered products and services.
Transparency drives user adoption
Explainability promotes trust and confidence among users and stakeholders. When AI-generated outputs are understandable, users feel more comfortable relying on the technology for critical decision-making. The ability to comprehend how a model arrives at its predictions builds transparency and accountability, fostering trust in AI systems.
Trust-Adoption Relationship:
Increased Transparency → Greater Trust → Higher Adoption Rates → Business Success
As a result, users are more likely to embrace and adopt AI technologies across various domains. This transparency doesn't just increase adoption; it strengthens the relationship between users and AI systems.
The connection between transparency and user adoption demonstrates the direct business value of investing in interpretable LLM implementations.
Practical approaches to AI transparency
Several practical approaches can enhance transparency in LLMs:
Such practical approaches allow users to better understand results without deciphering internal logic. These strategies differentiate products in the market while meeting growing expectations for explainable AI.
By implementing these approaches, product teams can create more trustworthy AI experiences that users feel comfortable adopting and relying upon.
Regulatory compliance through transparency
With increasing AI regulations worldwide, governments and organizations are focusing more on regulating AI deployment. Interpretability plays a crucial role in ensuring compliance with these regulations and ethical guidelines.
Transparency Benefits for Compliance:
- 1Demonstrates adherence to legal standards
- 2Shows commitment to ethical AI principles
- 3Protects user privacy and rights
- 4Reduces regulatory risks
- 5Minimizes potential liabilities
By providing insights into the decision-making process of LLMs, developers can demonstrate transparency and adherence to legal and ethical standards. Transparent AI systems not only protect users' privacy and rights but also pave the way for responsible and sustainable AI development.
This compliance aspect of interpretability creates a strong business case for transparency, as it reduces regulatory risks and potential liabilities.
Balancing performance with understandability
In AI, there's frequently a trade-off between model complexity (and thus accuracy) and interpretability. While simpler models are more straightforward to understand, they may not capture the nuances that complex systems like LLMs do.
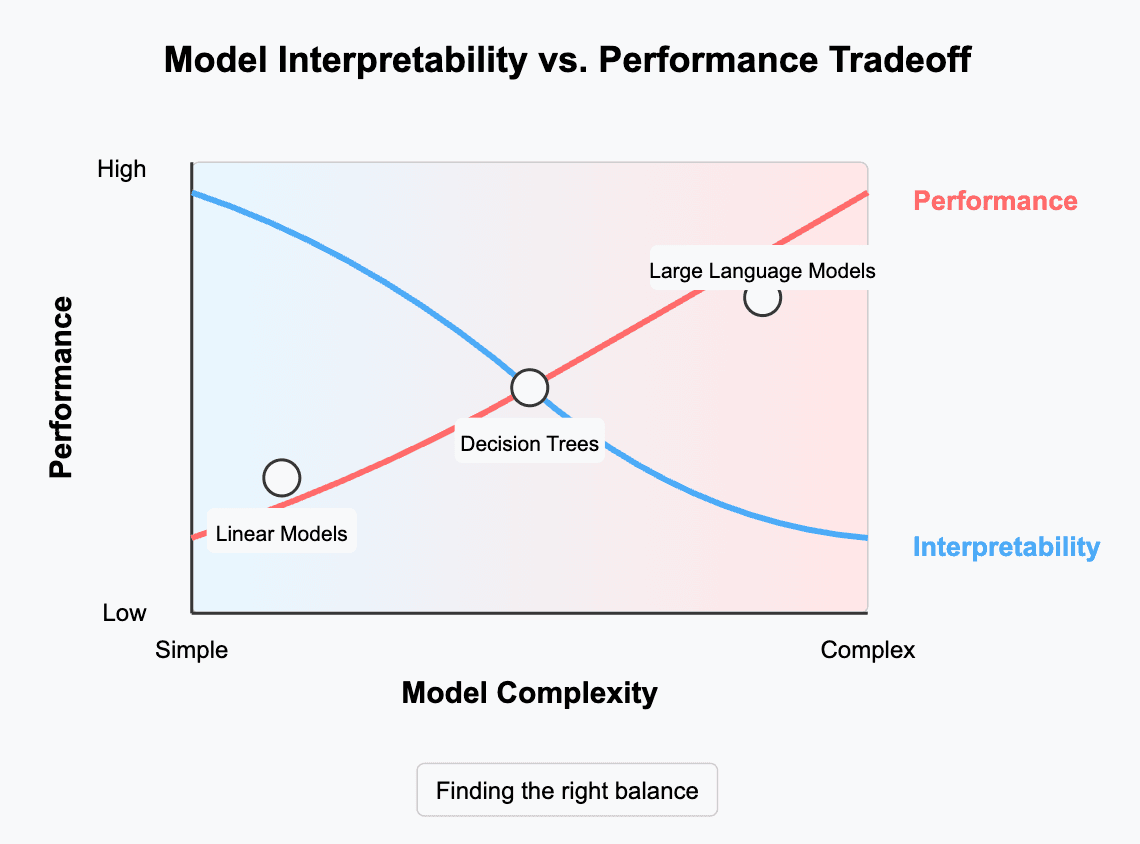
The Interpretability-Performance Spectrum
Finding the equilibrium is crucial for creating AI that is both efficient and comprehensible. The success of LLMs will be judged not just on their abilities but also on how easily they can be understood by users and those impacted by their decisions.
This balance ensures that recommendations align with the client's best interests while adhering to ethical guidelines.
The challenge of balancing performance with understandability requires thoughtful product decisions that prioritize transparency in critical areas while maintaining competitive capabilities.
The path to transparent AI
Organizations should invest in techniques and tools that enhance model interpretability. When implementing LLMs, businesses should:
- 1Prioritize clear explanation mechanisms from the design phase
- 2Identify high-risk decisions requiring transparency
- 3Implement layered explanations for different user types
- 4Develop metrics aligned with specific product goals
Implementation Framework:
Design Phase → Risk Assessment → Explanation Layers → Metrics Development → Continuous Improvement
Striking the right balance between complexity and understandability is not just a technical challenge but a core element of creating AI systems that are both reliable and effective.
Human-centered design approaches in LLM development will ensure these powerful tools serve their purpose while maintaining the transparency users need to trust and effectively collaborate with AI.
With user trust established as a critical factor, let's examine the specific regulatory requirements that govern LLM transparency across different regions and industries.
Regulatory compliance: Technical requirements for LLM transparency
Ensuring regulatory compliance for Large Language Models (LLMs) has become a critical consideration for organizations operating in highly regulated industries. As AI systems grow increasingly complex, stakeholders demand greater transparency into how these models function and make decisions.
Foundational transparency requirements
Technical transparency in LLMs requires implementing frameworks that document decision processes in detail. These frameworks must capture how models process inputs, weigh information, and generate outputs in human-understandable formats. Organizations need architectural patterns that enable continuous validation of LLM reasoning, allowing both internal teams and regulators to audit model behavior.
Regulatory Approaches by Region:
Different jurisdictions have varying approaches to AI explainability requirements. The EU AI Act stands at the forefront, mandating specific levels of transparency based on an application's risk level. High-risk LLM systems—like those used in healthcare, lending decisions, or legal advice—face the strictest requirements for explainability.
Understanding these foundational requirements is essential for product teams developing LLMs that will operate within regulated environments across multiple jurisdictions.
Implementation challenges in regulated environments
The complexity of LLMs creates significant technical hurdles for transparency. Many operate as "black boxes," making their decision-making processes difficult to interpret. This presents compliance issues in sectors where accountability is legally mandated.
Key Implementation Challenges:
- Balancing model performance with explainability
- Managing trade-offs between sophistication and transparency
- Translating technical concepts for non-technical stakeholders
- Meeting varying requirements across jurisdictions
- Retrofitting transparency into existing systems
Organizations must balance model performance with explainability requirements. There's often a trade-off between model sophistication and transparency. Too much focus on explainability can compromise an LLM's capabilities, while optimizing solely for performance may create regulatory risks.
Developers face the additional challenge of explaining technical concepts to non-technical stakeholders. This requires translating complex mechanisms into understandable terms without sacrificing accuracy.
These implementation challenges highlight why transparency must be considered from the earliest stages of product development rather than added retroactively.
Technical solutions for compliance
Some organizations are building explainability features directly into model architecture rather than treating them as afterthoughts. This approach integrates transparency from the design phase.
Compliance-Focused Development Practices:
- 1Implementing robust documentation systems
- 2Tracking model decisions systematically
- 3Maintaining clear records of inputs and outputs
- 4Documenting reasoning chains for audit purposes
- 5Combining explainable models for regulated decisions
Compliance-focused AI development practices include implementing robust documentation systems that track model decisions. These systems maintain clear records of inputs, outputs, and reasoning chains for audit purposes.
Hybrid approaches are gaining popularity, combining more explainable models for regulated decisions with complex models for less sensitive tasks. This balanced strategy maintains compliance while preserving advanced capabilities where appropriate.
These technical solutions provide practical approaches for product teams seeking to develop compliant LLM applications in regulated industries.
Cross-industry implementation frameworks
Technical implementation varies by industry. Financial regulators focus heavily on explainability for AI systems making lending or investment decisions. Healthcare regulations emphasize transparency in diagnostic applications, with the FDA's framework requiring explainable AI for patient safety.
Industry-Specific Requirements:
These sector-specific requirements create practical deployment challenges. Organizations may need to develop region-specific model variations to comply with differing standards across jurisdictions. Documentation requirements for model decisions may limit the use of fully opaque AI systems in regulated contexts.
The emerging standard involves compliance-focused development practices that address transparency from the outset of model design. This approach ensures systems remain auditable throughout their lifecycle.
Understanding these industry-specific frameworks helps product teams tailor their interpretability approaches to the particular regulatory requirements they face.
Future regulatory developments
Looking ahead, regulatory requirements for AI explainability will likely become more stringent and widespread. Organizations must develop governance frameworks that can adapt to this evolving landscape while maintaining the benefits of advanced AI capabilities.
Emerging Compliance Initiatives:
- COMPL-AI: Providing measurable, benchmark-driven methods for assessing compliance
- Safety-focused evaluation workflows
- Transparency measurement standards
- Ethical consideration frameworks
- Human oversight integration guidelines
Recent initiatives like COMPL-AI represent a significant advancement in aligning AI models with regulatory standards. By providing measurable, benchmark-driven methods for assessing compliance, these tools help developers address safety, transparency, and ethical considerations directly within evaluation workflows.
Human oversight will remain essential, as full transparency in LLMs may never be completely achievable. The most effective compliance strategies will combine technical solutions with robust human governance.
With a solid understanding of the regulatory landscape, let's examine specific implementation methods for creating interpretable LLMs.
Implementation methods for interpretable LLMs
Chain-of-thought prompting
Chain-of-thought prompting significantly enhances reasoning transparency in LLMs. This technique presents the model with examples of reasoning chains, allowing it to learn and apply similar patterns to complex problems. By incorporating intermediate reasoning steps, LLMs can more effectively tackle arithmetic, common sense, and symbolic reasoning tasks. This approach provides a window into the model's decision-making process, making its outputs more transparent and verifiable.
Chain-of-Thought Implementation Process:
Input Query → Example Reasoning Chain → Model Processing → Intermediate Steps → Final Output with Reasoning
When implemented in production environments, chain-of-thought prompting allows developers to observe how the model arrives at conclusions rather than just seeing the final answer.
This implementation method has proven particularly effective for creating transparent reasoning processes that users can follow and verify.
Retrieval-augmented generation
Retrieval-augmented generation (RAG) enables source verification by connecting LLM outputs to specific reference materials. This implementation method fetches relevant information from external knowledge bases and incorporates it into the generation process. The architecture typically involves:
- 1A retrieval component that identifies relevant documents
- 2A generation component that produces outputs using both the context and retrieved information
- 3Source attribution mechanisms that link generated content to specific references
RAG Architecture Components:
Query → Retrieval System → Knowledge Base → Relevant Documents → Generation System → Attributed Output
RAG significantly enhances interpretability by allowing users to trace information back to its source.
This approach addresses both factual accuracy and transparency, creating a clear connection between model outputs and authoritative sources.
Hybrid architectures
Combining interpretable modules with black-box components creates a balanced architecture that preserves performance while improving transparency. This approach involves:
Hybrid System Components:
- Using simpler, more interpretable models for critical decision points
- Employing black-box LLMs for generating creative or complex content
- Creating interfaces between components that expose internal reasoning
- Implementing logging systems that capture intermediate outputs
These hybrid systems allow organizations to maintain the performance advantages of sophisticated LLMs while providing transparency where it matters most.
This pragmatic approach recognizes that not all aspects of an LLM system require the same level of interpretability, focusing transparency efforts where they deliver the most value.
Performance considerations
Different interpretability techniques impact inference speed and cost in various ways:
Performance Impact by Technique:
- Chain-of-thought prompting increases token count and processing time by 30-50%
- RAG systems require additional infrastructure for document storage and retrieval
- Hybrid architectures may introduce communication overhead between components
When implementing interpretable LLMs, developers must balance the need for transparency with performance requirements and budget constraints.
Understanding these performance implications helps product teams make informed decisions about which interpretability methods best suit their specific use cases.
Evaluation metrics
Measuring explanation quality and consistency requires specialized metrics beyond standard performance indicators. Effective evaluation approaches include:
Evaluation Framework:
- 1Faithfulness assessment: measuring how accurately explanations reflect the model's actual reasoning
- 2Human judgment studies: gathering feedback on explanation clarity and usefulness
- 3Consistency tracking: evaluating whether similar inputs produce similar explanations
- 4Task performance correlation: determining if better explanations correlate with improved outcomes
Measurement Process:
Baseline Metrics → Implementation → Continuous Monitoring → Quality Assessment → Refinement
Organizations should establish baseline metrics before implementation and continuously monitor explanation quality over time.
Interpretability methods must be carefully selected based on the specific use case, regulatory requirements, and performance needs. The most successful implementations typically combine multiple approaches to create comprehensive transparency frameworks.
Conclusion
LLM interpretability represents more than a technical implementation detail—it's a strategic imperative for product success. As we've explored, transparent AI systems build user trust, simplify regulatory compliance, and provide competitive advantages in increasingly scrutinized markets.
Key Takeaways for Different Roles:
Product teams should prioritize interpretability from the design phase rather than treating it as an afterthought. Techniques like chain-of-thought prompting, retrieval-augmented generation, and hybrid architectures offer practical paths forward without sacrificing performance. The most effective approach often combines multiple methods tailored to specific product requirements.
For product managers, interpretability directly impacts adoption rates and customer retention. Consider building transparency features directly into your roadmap and positioning them as key differentiators in your market.
AI engineers should evaluate the performance trade-offs of different interpretability techniques, selecting frameworks that balance transparency with computational efficiency for your specific use case.
For startup leadership, interpretable AI represents an opportunity to establish trust advantages over competitors while reducing regulatory and reputation risks. As AI regulations continue to evolve, organizations that master interpretability now will be better positioned for sustainable growth.